Stable Video 3D makes a shocking debut: a single image generates 3D video without blind spots, and model weights are opened
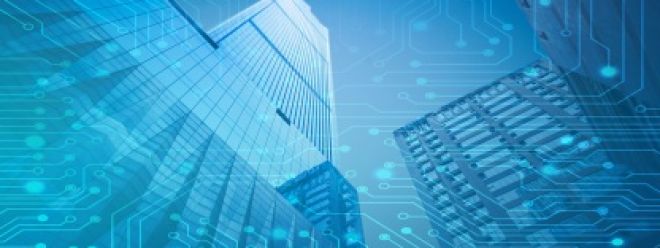
Stability AI has a new member in its large model family.
Yesterday, after launching Stable Diffusion and Stable Video Diffusion, Stability AI brought a large 3D video generation model "Stable Video 3D" (SV3D) to the community.
Currently, Stable Video 3D supports both commercial use, which requires joining the Stability AI membership (Membership); and non-commercial use, where users can download the model weights on Hugging Face.
Stability AI provides two model variants, namely SV3D_u and SV3D_p. Among them, SV3D_u generates orbital video based on a single image input and does not require camera adjustment; SV3D_p extends the generation capabilities by adapting a single image and orbital perspective, allowing the creation of 3D videos along a specified camera path.
Currently, the research paper on Stable Video 3D has been released, with three core authors.
- Paper address: https://stability.ai/s/SV3D_report.pdf
- Blog address: https://stability.ai/news/introducing-stable-video-3d
- Huggingface address: https://huggingface.co/stabilityai/sv3d
Technology overview
Stable Video 3D delivers significant advances in 3D generation, especially novel view synthesis (NVS).
Previous methods often tend to solve the problem of limited viewing angles and inconsistent inputs, while Stable Video 3D is able to provide a coherent view from any given angle and generalize well. As a result, the model not only increases pose controllability but also ensures consistent object appearance across multiple views, further improving key issues affecting realistic and accurate 3D generation.
As shown in the figure below, compared with Stable Zero123 and Zero-XL, Stable Video 3D is able to generate novel multi-views with stronger details, more faithfulness to the input image, and more consistent multi-viewpoints.
Additionally, Stable Video 3D leverages its multi-view consistency to optimize 3D Neural Radiance Fields (NeRF) to improve the quality of 3D meshes generated directly from new views.
To this end, Stability AI designed a masked fractional distillation sampling loss that further enhances the 3D quality of unseen regions in the predicted view. At the same time, to alleviate baked lighting problems, Stable Video 3D uses a decoupled lighting model that is optimized with 3D shapes and textures.
The image below shows an example of improved 3D mesh generation through 3D optimization when using the Stable Video 3D model and its output.
The figure below shows the comparison between the 3D mesh results generated by Stable Video 3D and those generated by EscherNet and Stable Zero123.
Architectural details
The architecture of the Stable Video 3D model is shown in Figure 2 below. It is built based on the Stable Video Diffusion architecture and contains a UNet with multiple layers. Each layer contains a residual block sequence with a Conv3D layer, and two A transformer block with attention layers (spatial and temporal).
The specific process is as follows:
(i) Delete the vector conditions of "fps id" and "motion bucket id" because they have nothing to do with Stable Video 3D;
(ii) The conditional image is embedded into the latent space through the VAE encoder of Stable Video Diffusion and then connected to the noisy latent state input zt at the noisy time step t leading to UNet;
(iii) The CLIPembedding matrix of the conditional image is provided to the cross-attention layer of each transformer block to serve as keys and values, and the query becomes the feature of the corresponding layer;
(iv) The camera trajectory is fed into the residual block along the diffusion noise time step. The camera pose angles ei and ai and the noise time step t are first embedded into the sinusoidal position embedding, then the camera pose embeddings are concatenated together for linear transformation and added to the noise time step embedding, and finally fed into each residual block and is added to the input features of the block.
In addition, Stability AI designed static orbits and dynamic orbits to study the impact of camera posture adjustment, as shown in Figure 3 below.
On a static orbit, the camera rotates around the object at equidistant azimuth angles using the same elevation angle as the condition image. The disadvantage of this is that based on the adjusted elevation angle, you may not get any information about the top or bottom of the object. In a dynamic orbit, the azimuth angles can be unequal, and the elevation angles of each view can also be different.
To build a dynamic orbit, Stability AI samples a static orbit, adding small random noise to its azimuth and a randomly weighted combination of sinusoids of different frequencies to its elevation. Doing so provides temporal smoothness and ensures that the camera trajectory ends along the same azimuth and elevation loop as the condition image.
Experimental results
Stability AI evaluates Stable Video 3D composite multi-view effects on static and dynamic orbits on unseen GSO and OmniObject3D datasets. The results are shown in Tables 1 to 4 below. Stable Video 3D achieves SOTA results in novel multi-view synthesis.
Tables 1 and 3 show the results of Stable Video 3D and other models on static orbits, showing that even the model SV3D_u without pose adjustment performs better than all previous methods.
Ablation analysis results show that SV3D_c and SV3D_p are better than SV3D_u in the generation of static trajectories, although the latter is trained exclusively on static trajectories.
Tables 2 and 4 below show the generation results of dynamic orbits, including pose adjustment models SV3D_c and SV3D_p, the latter achieving SOTA on all indicators.
The visual comparison results in Figure 6 below further demonstrate that Stable Video 3D generates images with greater detail, more faithfulness to conditional images, and more consistency across multiple viewing angles than previous work.
Please refer to the original paper for more technical details and experimental results.