340B narrowly beats 70B, Kimi robot "taunts" Nvidia's new open source model
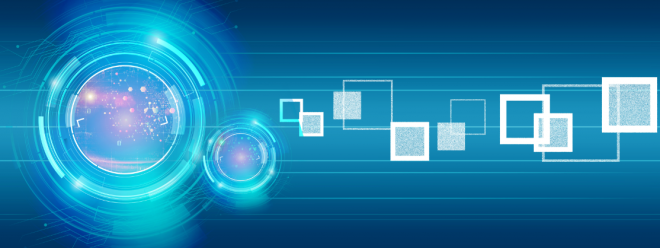
Ranking higher than Llama-3-70B , NVIDIA Nemotron-4 340B becomes the strongest open source model in the arena!
A few days ago, NVIDIA suddenly open-sourced the 340 billion parameter version of its general-purpose large model Nemotron.
Just recently, the arena updated the rankings:
The win rate heat map shows that Nemotron-4 340B has a win rate of 53% against Llama-3-70B .
How does Nemotron-4 340B perform? Let’s take a look.
New model latest results
To briefly review, NVIDIA suddenly announced the open source Nemotron-4 340B last Friday. The series includes basic models, instruction models, and reward models for generating synthetic data for training and improving LLM.
Nemotron-4 340B immediately topped the Hugging Face RewardBench list upon its release !
Next, Arena recently announced a series of evaluation results of Nemotron-4 340B.
In long text queries (length >= 500 tokens), Nemotron-4 340B ranks fifth, surpassing mainstream open source models such as Claude 3 Sonnet and Qwen 2-72B.
In terms of handling hard prompts , Nemotron-4 340B surpassed Claude 3 Sonnet and Llama3 70B-Instruct, showing its superior ability in coping with complex and difficult queries.
In the overall performance evaluation , Nemotron-4 340B's score and stability were both at an upper-middle level, surpassing many well-known open source models.
To summarize , Nemotron-4 340B has achieved good results, directly surpassing Mixtral 8x22B, Claude sonnet, Llama3 70B, Qwen 2, and sometimes even competing with GPT-4.
In fact, this model has appeared in the large model arena LMSys Chatbot Arena before, and its alias at that time was june-chatbot .
Specifically, this model supports 4K context windows, more than 50 natural languages, and more than 40 programming languages, and the training data is up to June 2023.
In terms of training data, NVIDIA uses up to 9 trillion tokens, of which 8 trillion are used for pre-training and 1 trillion are used for continued training to improve quality.
At BF16 precision, the model inference requires 8 H200s, or 16 H100/A100 80GB. If it is at FP8 precision, only 8 H100s are needed.
It is worth mentioning that the training of the instruction model is done on 98% synthetic data .
Synthetic data is undoubtedly the biggest highlight of Nemotron-4 340B, which has the potential to completely change the way LLM is trained.
Synthetic data is the future
Faced with the latest rankings, excited netizens suddenly sensed something was wrong:
Using 340B against 70B, and winning by a narrow margin, this is a bit unreasonable!
Even the robot Kimi turned on the "ridicule" mode:
Nvidia's operation has parameters as large as the universe, but its performance is on par with Llama-3-70B. It is a "big and small" in the technology world!
In this regard, Oleksii Kuchaiev, who is responsible for AI model alignment and customization at NVIDIA, came up with a key magic weapon:
Yes, the Nemotron-4 340B is commercially friendly and supports the generation of synthetic data .
Somshubra Majumdar, senior deep learning research engineer, praised this:
You can use it (for free) to generate all the data you want
This breakthrough marks an important milestone in the AI industry .
From now on, all walks of life no longer need to rely on large amounts of expensive real-world data sets. Using synthetic data, powerful domain-specific LLMs can be created!
So, how did NVIDIA achieve this?
In a nutshell, this is related to its reward model that is usually not released for open source.
Generating high-quality synthetic data requires not only excellent guidance models but also data screening according to specific needs.
Typically, the same model is used as the rater (LLM-as-Judge); however, in certain cases, it is more appropriate to use a dedicated reward model (Reward-Model-as-Judge) for evaluation.
The Nemotron-4 340B instruction model can generate high-quality data, and then the reward model can filter out data with multiple attributes.
It scores responses based on five attributes: usefulness, correctness, consistency, complexity, and verbosity .
Additionally, researchers can customize the Nemotron-4 340B base model using their own dedicated data in combination with the HelpSteer2 dataset to create their own command or reward models.
Going back to the battle with Llama-3-70B at the beginning, Nemotron-4 340B has a more relaxed permission, and perhaps this is its true value.
After all, data shortage has long been a common pain point in the industry.
Pablo Villalobos, an AI researcher at the Epoch Institute, predicts that there is a 50% chance that demand for high-quality data will outstrip supply by mid-2024 , and a 90% chance that this will happen by 2026.
New forecasts show that the risk of shortages will be delayed until 2028.
Synthetic data is the future and is gradually becoming an industry consensus...
Model address: https://huggingface.co/nvidia/Nemotron-4-340B-Instruct