How to govern data in the era of big models?
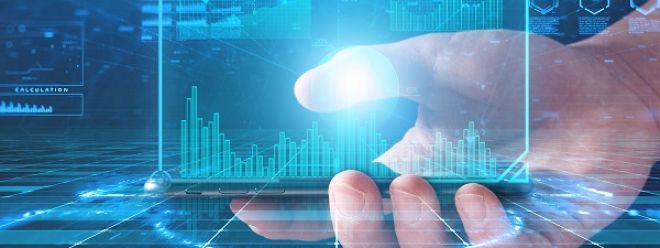
With the rapid development of ChatGPT and other large language models (LLMs), AI has become an indispensable part of our work and life, and has gradually evolved from simple text generation to advanced AI systems that can handle complex semantic understanding and generation.
The expansion of the capabilities and application scope of these models not only marks the advancement of technology, but also indicates that they have gradually moved from a supporting role to the center stage in actual business.
1. Evolution and upgrade of large models
General large models are usually trained based on large and diverse data sets, and have strong versatility and the ability to adapt to a wide range of application scenarios.
When these models are applied to specific industries (such as finance, healthcare, or law), they need to be further adjusted and optimized to suit specific business needs.
These are industry vertical big models - they are reduced and adjusted based on the big model framework. The models have fewer parameters, but are trained with industry-specific data sets and can achieve better performance in specific fields.
In specific areas, such as corporate law, by conducting detailed model fine-tuning and training on professional data sets, industry vertical big models can produce amazing results in such highly specialized fields. For example, by embedding them in the contract management system, during the contract approval process, AI assistants can assist in completing risk warning analysis of contract terms and help legal personnel identify problems more efficiently.
By continuously learning from large amounts of data, these industry-vertical models can not only understand the surface text of the language, but also grasp its deep context and emotions, providing a more accurate user interaction experience.
By combining specific knowledge from different business fields and industries, we have made significant breakthroughs in intelligent customer service, video image generation, precision marketing, biomedical research, and complex financial market forecasting.
2. Data requirements for training large vertical models in an industry
High-quality data is very important for training large vertical models in an industry.
Its core requirements include data accuracy, completeness, representativeness, unbiasedness, and proper preprocessing. The dataset needs to be accurate and cover a wide range of scenarios and situations to ensure that the model can generalize to new environments. Diversity is also key, which means that the dataset should cover different languages, fields, cultures, and backgrounds.
Preprocessing and feature engineering of high-quality data is another key step to improve model accuracy. Proper data formatting and structuring are required so that the model can read and process the data effectively. In addition, it is also important to deal with noise and outliers in the data, as these factors may interfere with the model's learning process.
In the data preparation stage, incorrect data labeling or inaccurate data classification will directly affect the training effect of the model. For example, the accuracy of text data labels, the accuracy of automatic topic identification, the clarity of industry classification, and data denoising are all important steps to ensure the quality of the data set.
The integration and management of multimodal data sets are also gaining more and more attention. Vertical large models may involve the processing of multiple data types such as text, images, and voice. Effective data processing requires the integration of these different types of data, automatic identification, classification, and association with other data types to support more complex AI applications.
3. Data governance issues in industry vertical large model training
Large vertical models are expensive to train and maintain, and have complex technical requirements.
In the application and development of large vertical models in the industry, data governance faces many challenges. If these problems are not handled properly, they will not only affect the performance of the model, but are also likely to cause legal and ethical disputes.
Here are a few key data governance issues.
- Data privacy and security: As the scale of data increases, protecting personal privacy and data security has become a major challenge. The data sets required to train large models may contain sensitive information, such as personal identity details, behavioral data, etc., which may lead to privacy leaks if not properly processed.
- Data quality and consistency: Inconsistent data, incorrect annotations, or incomplete information can seriously affect the training quality of large models and the accuracy of results. Poor quality data may lead to model deviation or even make it completely unusable in real scenarios.
- Data bias and fairness: Data sets may contain biases that reflect the non-objectivity of data collection. For example, a data set may be biased towards a particular gender, race, or social group, causing the model to replicate or even amplify these biases in actual applications, affecting the fairness of decision-making.
- Data size and processing capacity: Large models require a large amount of data for training, which places higher demands on data processing and storage. The management, storage and processing of large-scale data not only requires high technical requirements, but also is costly.
4. Solution framework for effective data governance
In the data governance of industry vertical big models, a comprehensive and detailed solution framework is very important.
First, data collection, storage, processing and analysis must meet high standards of quality control to ensure the accuracy, consistency and integrity of the data.
Due to the complexity of vertical large-scale model training and the diversity of data requirements, we need to establish a multi-level data governance strategy to address these challenges.
An effective data governance solution should include the following aspects.
- Data collection and preprocessing: For different types of data, precise preprocessing processes are implemented, including data cleaning, denoising, standardization, and vectorization. This step is crucial to improving data availability and the efficiency of model training.
- Annotation and fine-tuning framework: Annotation guidelines and formats customized for specific domains and tasks ensure consistency and standardization of data annotation. In addition, specialized datasets are provided for model fine-tuning and domain adaptation development, such as domain-specific question-answering sets or sentiment analysis data.
- Comprehensive evaluation and testing: Build test evaluation data sets that are suitable for different application scenarios to verify the performance and adaptability of the model. This not only helps evaluate the actual application effect of the model, but also serves as the basis for continuous optimization of the model.
- Legal and Compliance Compliance: Ensure that all data processing activities comply with relevant laws, regulations, copyrights and ethical standards. This not only involves the legal collection and use of data, but also includes the secure storage and processing of data to prevent data leakage or abuse.
- Data lifecycle management: Develop a comprehensive data lifecycle management strategy from data generation, storage, use to disposal. This includes data archiving, processing and reuse, version control, quality inspection, tracking and measurement, and backup and recovery to support the continuity and systematicness of data governance.
5. From industry-specific big models to enterprise-specific big models
The further development trend of industry vertical big models will be the emergence of enterprise-specific big models.
At present, some leading companies have begun to try to absorb the results of vertical big models and train company-specific big models based on their own data and knowledge in order to create unique competitive advantages.
We should see that whether it is a general big model, a vertical big model customized for a specific industry, or an enterprise-specific big model, their effectiveness and efficiency are largely limited by the quality of the training data.
The creation of enterprise-specific large models is more sophisticated and requires higher accuracy.
Before building an enterprise-specific big model, the enterprise needs to complete internal data governance.
This not only involves data collection and storage, but also data cleaning, standardization, security protection and compliance checking.
Especially when handling sensitive data, strict data governance processes can prevent data leakage and abuse and protect the interests of enterprises and customers.
In addition, the complexity of data governance lies in the fact that it is far from a purely technical task. Effective data quality is inseparable from the guarantee of data management system and data governance process.