The most powerful open source multi-modal generation model MM-Interleaved: the first feature synchronizer
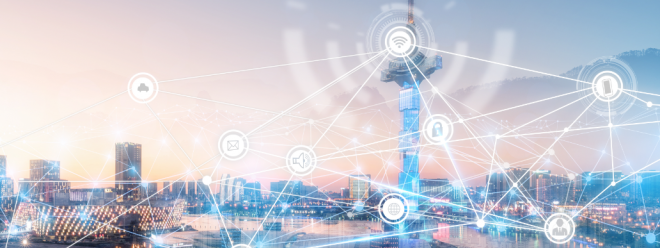
Imagine that AI can not only chat, but also has "eyes", can understand pictures, and can even express itself through drawing! This means that you can chat with them, share pictures or videos, and they can also respond to you with pictures and texts.
Recently, Shanghai Artificial Intelligence Laboratory, together with the Chinese University of Hong Kong Multimedia Laboratory (MMLab), Tsinghua University, SenseTime, University of Toronto and many other universities and institutions, jointly released a versatile and strongest open source multi-modal generation model MM- Interleaved refreshes multi-task SOTA with the newly proposed multi-modal feature synchronizer. It has the ability to accurately understand high-resolution image details and subtle semantics, supports arbitrarily interspersed image and text input and output, and brings a new breakthrough in multi-modal generation of large models.
Paper address: https://arxiv.org/pdf/2401.10208.pdf
Project address: https://github.com/OpenGVLab/MM-Interleaved
Model address: https://huggingface.co/OpenGVLab/MM-Interleaved/tree/main/mm_interleaved_pretrain
MM-Interleaved can easily write engaging travel diaries and fairy tales, accurately understand robot operations, and even analyze the GUI interfaces of computers and mobile phones, and create unique and beautiful pictures. It can even teach you how to cook, play games with you, and become a personal assistant ready for command at any time! Without further ado, let’s just look at the effects:
Easily understand complex multimodal contexts
MM-Interleaved can independently reason based on the picture and text context to generate text responses that meet the requirements. It can also calculate fruit math problems:
You can also use common sense to deduce the company corresponding to the logo image and introduce it:
It can also accurately recognize handwritten text marked with a red circle:
In addition, the model can also directly understand robot actions represented by sequence images:
And how to build a fence in Minecraft such game operations:
It can even teach users step-by-step how to configure grayscale on the mobile UI interface based on the context:
And precise positioning to find the plane hidden behind:
Use your imagination to generate images of different styles
The MM-Interleaved model can also perform various complex image generation tasks well. For example, generate a silhouette of a grand piano based on the detailed description provided by the user:
Or when the user specifies the objects or styles that the generated image should contain in multiple forms, the MM-Interleaved framework can also easily handle it.
For example, generate a watercolor-style elephant:
Generate a drawing of a cat in the style of a dog:
A wooden house among sunflowers:
And when generating ocean wave images, the corresponding style is intelligently inferred based on the context.
Image generation takes into account spatial consistency
What’s even more surprising is that MM-Interleaved also has the ability to generate images based on the input segmentation map and corresponding text description, and ensure that the generated image and the segmentation map are consistent in spatial layout.
This feature not only demonstrates the model's excellent performance in image and text generation tasks, but also provides users with a more flexible and intuitive operating experience.
Independently generate articles with pictures and texts
In addition, just by providing a simple beginning, MM-Interleaved can continue writing independently to generate semantically coherent, illustrated and text-rich articles on a variety of topics.
Whether it's a fairy tale about a rose:
Tutorial guide to teach you how to make apple juice:
Or a plot fragment from a cartoon animation:
MM-Interleaved frameworks all demonstrate outstanding creativity. This makes the MM-Interleaved framework an intelligent collaborator with unlimited creativity, helping users easily create engaging graphic and text works.
MM-Interleaved is committed to solving the core problems in the training of large multi-modal models with interleaved graphics and text, and proposes a new end-to-end pre-training framework through in-depth research.
The model trained based on MM-Interleaved, with fewer parameters and no private data, not only performs well on multiple zero-shot multi-modal understanding tasks, but is ahead of the latest research work at home and abroad, such as Flamingo, Emu2, etc. .
It can also be further used in visual question answering (VQA), image caption, referring expression comprehension, segment-to-image generation, and visual storytelling through supervised fine-tuning. ) and achieve better comprehensive performance on multiple downstream tasks.
At present, the pre-training weights of the model and the corresponding code implementation have been open sourced on GitHub.
Multimodal feature synchronizer joins hands with new end-to-end training framework
MM-Interleaved proposes a new end-to-end training framework specifically for image and text interleaved data.
The framework supports multi-scale image features as input and does not add any additional constraints to the intermediate features of images and texts. Instead, it directly adopts the self-supervised training goal of predicting the next text token or the next image to achieve single-stage unified pre-training. paradigm.
Compared with previous methods, MM-Interleaved not only supports interleaved generation of text and images, but also efficiently captures more detailed information in images.
In addition, the key implementation of MM-Interleaved also includes a universal multi-modal feature synchronizer.
The synchronizer can dynamically inject fine-grained features of multiple high-resolution images into multi-modal large models and image decoders, achieving cross-modal feature synchronization while decoding and generating text and images.
This innovative design enables MM-Interleaved to inject new vitality into the development of multi-modal large models.
Leading performance in multiple tasks
As shown in Tables 1 and 3, MM-Interleaved achieves excellent performance on both zero-shot multimodal understanding and generation tasks. This achievement not only demonstrates the powerful capabilities of the framework, but also highlights its strong versatility in dealing with diverse tasks.
Tables 2 and 4 show the experimental results of MM-Interleaved after further fine-tuning. Its performance is also very good in multiple downstream tasks such as reference understanding, image generation based on segmentation maps, and image and text interleaved generation.
This shows that MM-Interleaved not only performs well in the pre-training stage, but also maintains its leading position after fine-tuning for specific tasks, thus providing reliable support for the wide application of multi-modal large models.
in conclusion
The advent of MM-Interleaved marks a key step in the development of multi-modal large models towards achieving comprehensive end-to-end unified modeling and training.
The success of this framework is not only reflected in its excellent performance in the pre-training stage, but also in its overall performance on various specific downstream tasks after fine-tuning.
Its unique contribution not only demonstrates powerful multi-modal processing capabilities, but also opens up broader possibilities for the open source community to build a new generation of multi-modal large models.
MM-Interleaved also provides new ideas and tools for future processing of interleaved graphics and text data, laying a solid foundation for more intelligent and flexible graphics and text generation and understanding.
We look forward to seeing this innovation bring more surprises to related applications in more fields.