The top threat to AI: poisoning attacks
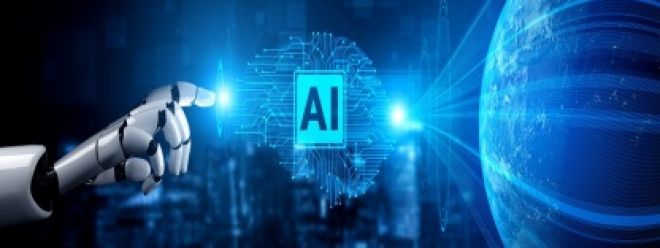
With Apple, a trillion-dollar company that controls the entrance to digital life, jumping into the artificial intelligence (AI) track, the curtain of the democratization of AI technology has officially been opened, and at the same time, the issue of AI security has been pushed to the forefront of public opinion.
According to a smartphone survey report released by UBS on Monday, only 27% of smartphone users outside of China are interested in devices that provide generative AI functions, and price and privacy are the issues that users are most concerned about. Obviously, users are most concerned about new threats to privacy and AI security rather than the efficiency and experience brought by AI.
The top threat to AI: poisoning attacks
Recently, the National Institute of Standards and Technology (NIST) of the United States issued a warning that with the rapid popularization of artificial intelligence technology, more and more hackers will launch "poisoning attacks" and the security of AI applications will face severe tests.
Poisoning attack is a malicious behavior against artificial intelligence (AI) systems, in which hackers influence the output of AI systems by manipulating input data or directly modifying models.
In the past, the industry did not pay much attention to "poisoning attacks" on AI systems. Software company Splunk once pointed out in its "2024 Security State Report": "AI poisoning is still a possibility, but it is not yet widespread."
But security experts are warning CISOs to be on high alert amid signs that hackers are increasingly targeting AI systems, particularly through poisoning attacks that corrupt data or models, and that companies of all sizes and types could be targets.
Consulting firm Protiviti revealed that one of its client companies recently suffered a poisoning attack: hackers attempted to manipulate the output of the company's AI system by feeding malicious input data.
“All organizations, whether they develop AI models in-house or use third-party AI tools, are at risk for poisoning attacks,” Protiviti noted.
Four main types of poisoning attacks
NIST highlighted the dangers of poisoning attacks in a January 2024 report: “Poisoning attacks are powerful enough to compromise the availability or integrity of AI systems.”
NIST classifies poisoning attacks into four categories:
- Availability poisoning: Indiscriminately affecting the entire machine learning model, equivalent to a denial of service attack against the AI system.
- Target poisoning: Hackers induce machine learning models to produce incorrect prediction results on a small number of target samples.
- Backdoor poisoning: By adding small trigger patches to a subset of images during training and changing their labels, an image classifier can be influenced to activate erroneous behaviors when used in the field.
- Model poisoning: Directly modifying a trained machine learning model to inject malicious functionality to make it behave abnormally under certain circumstances.
NIST and security experts point out that in addition to poisoning attacks, AI systems also face a variety of attacks such as privacy leakage and direct and indirect prompt injection.
"Enterprise deployment of AI introduces a whole new attack surface, and we've already seen exploits demonstrated by academics and other researchers," said Apostol Vassilev, director of the NIST research team. "As AI becomes more common, the value of the attacks increases, which is why we see more serious exploits, and we've seen an increase in related cases."
AI poisoning attacks can come from inside or outside
Security experts say poisoning attacks can be launched by both insiders and external hackers, similar to traditional cyber attacks.
David Youssef, managing director of FTI Consulting, said nation-state hackers are perhaps one of the biggest risks because they have the capability and resources to invest in these types of attacks.
Experts point out that hackers' motivations for launching AI poisoning attacks are similar to those for traditional cyber attacks, such as causing damage or loss, obtaining confidential data or extorting money.
Main target: AI manufacturers
While any organization using AI could become a victim, Kayne McGladrey, senior IEEE member and CISO of Hyperproof, said hackers are more likely to target tech companies that build and train AI systems.
A recent case exposed the potential huge risks upstream of the AI technology supply chain. Researchers at technology company JFrog found that about 100 malicious machine learning models were uploaded to the public AI model library HuggingFace.
The researchers noted that these malicious models could allow attackers to inject malicious code into user machines when the model is loaded, potentially quickly compromising a large number of user environments.
What should CISOs do?
According to a February survey by ISC2, many CISOs are not prepared to deal with AI risks. The report found that 75% of more than 1,100 respondents expressed moderate to extreme concerns that AI would be used for cyberattacks or other malicious activities, with deep fakes, false information and social engineering being the top three concerns of network professionals.
Despite this high level of concern, only 60% said they were confident they could lead their organizations in the safe adoption of AI. Additionally, 41% said they had little or no expertise in securing AI and ML technologies. Meanwhile, only 27% said their organizations had formal policies in place regarding the safe and ethical use of AI.
“The average CISO is not good at AI development and does not have AI skills as a core competency,” said Jon France, chief information security officer at ISC2.
Security experts suggest that defending against poisoning attacks requires a multi-layered defense strategy, including strong access and identity management programs, security information and event management (SIEM) systems, and anomaly detection tools. In addition, good data governance practices and monitoring and supervision of AI tools are also required.
NIST also provides detailed mitigation strategies and details about poisoning attacks in its report Adversarial Machine Learning.
Finally, some security leaders strongly recommend that CISOs add professionals with AI security training to their teams (normal SOC teams are not equipped to evaluate training datasets and AI models) and work with other executives to identify and understand the risks associated with AI tools (including poisoning attacks) and develop risk mitigation strategies.