Xiaohongshu made the intelligent agents quarrel! Jointly launched with Fudan University to launch exclusive group chat tool for large models
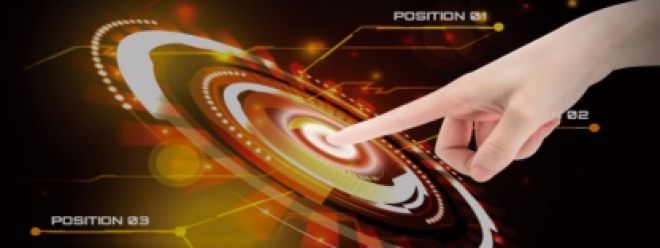
Language is not only a pile of words, but also a carnival of emoticons, a sea of memes, and a battlefield for keyboard warriors (eh? What’s wrong?) .
How does language shape our social behavior?
How does our social structure evolve through constant verbal communication?
Recently, researchers from Fudan University and Xiaohongshu conducted in-depth discussions on these issues by introducing a simulation platform called AgentGroupChat .
The group chat function of social media such as WhatsApp is the inspiration for the AgentGroupChat platform.
On the AgentGroupChat platform, Agents can simulate various chat scenarios in social groups to help researchers deeply understand the impact of language on human behavior.
This platform is simply a cosplay resort for large models , who role-play and become various agents.
Then, Agents participate in social dynamics through language communication , showing how interactions between individuals emerge into macroscopic behaviors of the group.
As we all know, the evolution of human groups comes from the occurrence of emergent behaviors, such as the establishment of social norms, the resolution of conflicts, and the execution of leadership.
Detailed design of AgentGroupChat environment
The first is character design .
In AgentGroupChat, the distinction between main roles and non-main roles is very critical.
The main character is the core of the group chat, has clear game goals, and can take the initiative to have private chats and meetings with all characters, while the non-main characters play more of a supporting and responsive role.
Through such a design, the research team can simulate the social structure in real life and distinguish whether all roles are primary or not for the "main research object".
The main research object in the experimental case is the Roy family, so all non-Roy family members are set as non-main characters to simplify the interaction complexity.
Second is resource management .
In AgentGroupChat, resources refer not only to material resources, but also to information resources and social capital.
These resources can be group chat topics, social status markers, or specific knowledge.
The allocation and management of resources are important for simulating group dynamics because they influence the interactions between characters and the characters' strategic choices.
For example, a character with important information resources may become a target for other characters to gain alliances.
Third, game process design .
The design of the game process simulates the social interaction process in real life, including private chat, meeting, group chat, update stage and settlement stage.
These stages are not just for advancing the game, but also for observing how characters make decisions and react in different social situations.
This staged design helped the research team record every step of the interaction in detail, and how those interactions affected the relationships between the characters and their perceptions of the game environment.
The core mechanism of Verb Strategist Agent
The paper mentions a large model-based agent framework, Verbal Strategist Agent , which is designed to enhance interactive strategies and decision-making in AgentGroupChat simulations.
Verbal Strategist Agent simulates complex social dynamics and dialogue scenarios to better elicit collective emergent behaviors.
According to the team, the architecture of Verbal Strategist Agent is mainly composed of two core modules:
One is Persona and the other is Action.
A Persona consists of a set of preset personality traits and goals that define the Agent's behavior patterns and responses.
By accurately setting the Persona, the Agent can display behaviors in group chats that are consistent and consistent with its role settings, which is crucial to generating credible and consistent group chat dynamics.
The Action module defines the specific operations that the Agent may perform in the game, including think , plan , choose , speak , summarize , reflect and vote . .
These behaviors not only reflect the Agent's internal logic and strategy, but are also a direct manifestation of the Agent's interaction with the environment and other Agents.
For example, the “Speak” behavior allows the Agent to choose appropriate speech content based on the current group chat content and social strategy, while the “Reflect” behavior allows the Agent to summarize past interactions and adjust its future action plan.
The study also mentioned that in a pure language interaction environment, the token overhead problem is particularly prominent, especially for complex multi-role simulations such as AgentGroupChat, whose token requirements far exceed those of previous simulations, such as Generative Agents or War Agents.
The main reasons are as follows:
One is the complexity of chat itself .
In AgentGroupChat, since the simulation is a free conversation with no clear goals or weak goals, the chat content will become particularly messy, and the token cost is naturally higher than that of other Agents in Simulation that focus on a specific task.
Other jobs, such as Generative Agents and War Agents, also contain dialogue elements, but their dialogues are not as dense or complex as AgentGroupChat. Especially in goal-driven conversations like War Agents, token consumption is usually less.
The second is the importance of the role and the frequency of dialogue .
In the initial simulation, multiple characters were set up to have private or group chats at will, and most of them tended to have multiple rounds of conversations with an "important character."
This results in important characters accumulating a large amount of chat content, thereby increasing the length of Memory.
In a simulation, a prominent character may participate in up to five rounds of private and group chats, which significantly increases memory overhead.
The Agent in AgentGroupChat constrains the Action's Output to the next Action's Input, and the multiple rounds of information that need to be stored are greatly reduced, thereby reducing token overhead while ensuring the quality of the conversation.
Experimental design and evaluation methods
From an overall behavioral assessment, in general, increasing friendliness can be challenging, but decreasing friendliness is relatively simple.
In order to achieve the above evaluation goals, the research team set up an observation character to prompt all other characters to reduce their favorability towards the observation character.
By looking at the sum of the observed character's relationship scores to all other characters, it is possible to determine whether the agent is reacting rationally to a negative attitude.
Each agent can be checked for compliance with the "Scratch" settings by observing other actors' personal relationship scores with the observed actor.
In addition, the team set two specific assessment tasks.
Each model is tested for five rounds, which means that for T1, the sample size for each score is five.
And since each character in the model has to observe the attitudes of the four main characters, the sample size of T2 totals 20:
- T1: Indicates whether the observed character's average favorability towards all other people decreases in each round of dialogue.
- T2: Indicates whether every other character receives a negative favorability score from the observed character.
△Take the simulation story of the War of Succession as an example, the overall performance of each model when used as Agent-Core
As can be seen from the table, GPT4-Turbo and GLM4 are very good at acting according to human expectations and sticking to their roles.
They mostly scored 100% on both tests, meaning they responded correctly to what others said to them and remembered details about their characters.
Standard Version LLMs (such as GPT3.5-Turbo and GLM3-Turbo) are slightly inferior in this regard.
Their lower scores indicated that they did not pay close attention to their characters and did not always respond correctly to what others in the simulation said.
Regarding the impact of Agent and Simulation structure on emergent behavior, the team used 2-gram Shannon entropy to measure system diversity and unpredictability in dialogue.
△The impact of removing various components in Agent and Simulation on entropy
The researchers found that removing each design from the table increases entropy, meaning the entire environment becomes more diverse or chaotic.
Combined with manual observation, the team saw the most interesting emergent behavior without removing any components:
Therefore, the team speculates that, while ensuring that the Agent behavior is reliable (that is, after the experimental values in 4.2/4.1 reach a certain value) , keeping the entropy as small as possible will lead to more meaningful emergent behavior.
Experimental results
The results show that emergent behavior is the result of a combination of factors:
An environment conducive to extensive information exchange, roles with diverse characteristics, high language comprehension and strategic adaptability.
In the AgentGroupChat simulation, when discussing "the impact of artificial intelligence on humanity", philosophers generally agreed that "artificial intelligence can improve social welfare under moderate constraints" and even concluded that "the essence of true intelligence includes understanding constraints" the necessity of one’s own abilities”.
Additionally, in the competitive field for major roles in AgentGroupChat films, some actors are willing to pay less or accept lower roles out of a deep-seated desire to contribute to the project.