With 4 trillion transistors, a single machine can train models 10 times larger than GPT4. The fastest and largest chip is available.
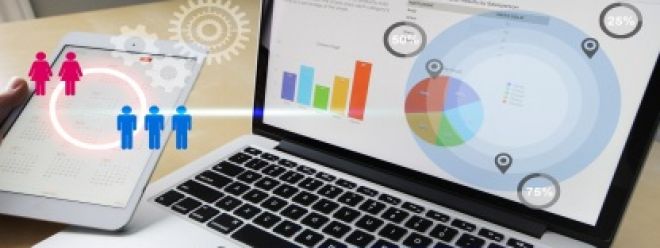
Cerebras has been developing "big" chips for a long time. The Wafer Scale Engine (WSE-1) they previously released is larger than the iPad. Although the second-generation WSE-2 has no change in area, it has an astonishing 2.6 trillion transistors and 850,000 AI-optimized cores.
The now launched WSE-3 contains 4 trillion transistors. At the same power consumption and price, WSE-3 has twice the performance of the previous record holder WSE-2.
The WSE-3 released this time is specially designed to train the industry's largest AI model. Based on 5 nanometers and 4 trillion transistors, WSE-3 will power the Cerebras CS-3 artificial intelligence supercomputer, through 900,000 artificial intelligence The intelligently optimized computing core provides a peak AI performance of 125 petaflops per second (1 petaflops refers to 1,000,000,000,000,000 (one trillion) floating point operations per second).
WSE-3 is square in shape with a side length of 21.5 cm (area 46225mm^2), and almost uses the entire 300 mm silicon wafer to manufacture a chip. It seems that with WSE-3, Cerebras can continue to produce the world's largest single chip.
What is the concept of large size of WSE-3? After comparing it with Nvidia H100 GPU, we found that the former is 57 times larger, the number of cores has increased by 52 times, the chip memory has increased by 800 times, and the memory bandwidth has increased by 7000 times. The structure Bandwidth has increased by more than 3700 times. These are the basis for the chip to achieve high performance.
Image source: https://spectrum.ieee.org/cerebras-chip-cs3
The following figure shows the characteristics of WSE-3:
WSE-3
Some parameters of the first two generations of wafer-level engines. Image source: https://twitter.com/intelligenz_b/status/1768085044898275534
CS-3 computers equipped with WSE-3 can theoretically handle large language models with 24 trillion parameters, which is an order of magnitude higher than the parameters of top generative AI models such as OpenAI's GPT-4 (rumored to have 1 trillion parameter). It seems that it is possible to run a model with 24 trillion parameters on a single machine.
Image source: https://www.servethehome.com/cerebras-wse-3-ai-chip-launched-56x-larger-than-nvidia-h100-vertiv-supermicro-hpe-qualcomm/
CS-3 has a huge memory system of up to 1.2 PB and is designed to train next-generation cutting-edge models that are 10 times larger than GPT-4 and Gemini. A 24 trillion parameter model can be stored in a single logical memory space without the need for partitioning or refactoring, greatly simplifying training workflows and increasing developer productivity. Training a 1 trillion parameter model on CS-3 is as easy as training a 1 billion parameter model on a GPU.
CS-3 is built for enterprise and hyperscale needs. A compact four-system configuration can fine-tune a 70B model in a day, while fully scalable with 2048 systems, the Llama 70B can be trained from scratch in a day, an unprecedented feat for generative AI.
The latest Cerebras software framework provides native support for PyTorch 2.0 and the latest AI models and technologies such as multimodal models, visual transformers, MoE, and diffusion models. Cerebras remains the only platform to offer native hardware acceleration for dynamic and unstructured sparsity, increasing training speed by up to 8x.
"Eight years ago, when we started this journey, everyone said wafer-scale processors were a pipe dream. We are extremely proud to launch the third generation of breakthrough AI chips and are excited to bring WSE-3 and CS-3 is brought to market to help solve today’s biggest artificial intelligence challenges,” said Andrew Feldman, CEO and co-founder of Cerebras.
Cerebras Co-Founder and CEO Andrew Feldman
Excellent power efficiency and software ease of use
Because every component is optimized for AI work, CS-3 delivers higher computing performance in less space and with lower power consumption than any other system. CS-3 doubles performance while maintaining the same power consumption.
The CS-3 offers exceptional ease of use. Compared with GPUs commonly used for large models, CS-3 requires 97% less code and can train models from 1B to 24T parameters in pure data parallel mode. A GPT-3 sized model requires only 565 lines of code to implement on Cerebras (versus 20,507 lines on GPU) - an industry record.
Image source: https://www.servethehome.com/cerebras-wse-3-ai-chip-launched-56x-larger-than-nvidia-h100-vertiv-supermicro-hpe-qualcomm/
Currently, Cerebras has accumulated a large backlog of orders from technology companies and scientific research institutions. Rick Stevens, deputy laboratory director for computing, environmental and life sciences at Argonne National Laboratory in the United States, praised: "Cerebras' bold spirit will pave the way for the future of artificial intelligence."