Edge Computing in 5G
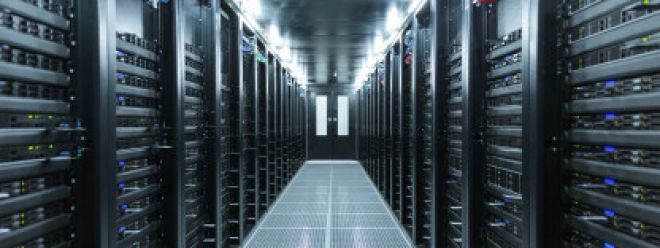
Edge Computing in 5G
01/ Introduction
Edge computing is a computing paradigm that enables small clouds or edge servers in edge clouds to boost cloud capabilities at the edge of the network to perform computationally intensive tasks and store large amounts of data near user equipment (UE). Due to the limited processing, computing, and storage capabilities of UEs, traditional cloud computing allows computing and storage of UEs in data centers. Therefore, traditional cloud computing is a centralized computing paradigm. Edge computing is well suited to the wireless communication needs of next-generation applications, such as augmented reality and virtual reality, which are interactive in nature. These highly interactive applications are computationally intensive, have high quality of service requirements, and can generate as much as 30.6 EB of data per month. The limited capabilities of terminals guarantee the needs of edge computing, including: receiving and storing large amounts of real-time data, processing computing and analyzing data, and making and distributing decisions on the local mini-cloud. So the edge servers in the mini cloud have the capabilities of the cloud, but at a different scale, they are on-premises, not in a remote data center, which might be far from the endpoint.
The content of this article mainly includes:
① An overview of 5G and edge computing, and answered a lot of questions about the use of edge computing in 5G;
② Classification of edge computing in 5G;
③ The most advanced edge computing solution in 5G;
④ Problems to be solved;
⑤ Summary of this article.
02 / Overview of 5G and edge computing
A. 5G system requirements
The 5G network can firstly generate a large amount of data for consumers to use; secondly, strict QoS requirements are required to meet highly interactive applications, and ensure ultra-low latency and high throughput; finally, it must support heterogeneous environments to satisfy different terminals, Interoperability of QoS requirements, network types, etc.
5G mainly consists of three emerging technologies. First, millimeter-wave communication uses high-frequency bands (30GHz-300GHz) to provide high bandwidth; secondly, small cell deployment allows terminals to use millimeter-wave communication to reduce interference and transmission distance; third, multiple-input multiple-output, or massive MIMO, allows base stations to use Large antennas enable directional transmissions, reducing interference while allowing adjacent nodes to communicate simultaneously. These emerging technologies enable 5G networks to have higher network capacity to supply more terminals.
B. Main features of 5G data
According to the time characteristics of the data, the data can be divided into three categories:
· Hard real-time data with strict predefined delays, such as data generated by games, healthcare;
Soft real-time data has a predefined delay, but some predefined and limited delays are acceptable, such as data generated by intelligent traffic signal control systems;
· Non-real-time data, latency is acceptable.
Edge computing is used to process applications and services with hard real-time requirements on edge servers, which can significantly reduce latency due to proximity to terminals. For applications and services with soft real-time requirements or limited end-to-end delay, if the response delay between the terminal and the cloud is higher than the demand, the task will be processed by the edge server, otherwise it will be offloaded to the cloud. Applications and services with non-real-time requirements can offload tasks to the cloud to achieve load balancing.
C. Significance of edge computing
Edge computing adopts a decentralized model, bringing cloud computing capabilities closer to terminals and reducing delays. The models of cloud computing and edge computing are shown in Figure 1. Cloud servers are usually located in the core network, and edge servers are located at the edge of the network. Edge computing can operate not only as a single computing platform, but also as a platform that operates in collaboration with other components. At the same time, edge computing makes up for the problem that cloud computing is not suitable for applications with large amount of calculation, strong interaction and high QoS requirements, reduces energy consumption, and also meets the sub-millisecond requirements of 5G applications.
Figure 1 Cloud Computing and Edge Computing Models
D. Key requirements for deploying edge computing in 5G
Key requirements for deploying edge computing in 5G include the following:
Realize real-time interaction and reduce latency;
Perform local data processing and user request processing on the edge server to reduce traffic transmission between the cell and the core network ;
Embed the edge server into the base station to achieve convenient access to the cloud, and use the millimeter wave frequency band to achieve high-speed data transmission ;
Ensure high availability of edge cloud services to ensure that services provided at the edge can be continuously and stably provided to users.
E. Application of edge computing in 5G environment
Many applications in 5G will rely on edge computing for real-time interaction, local processing, high data rates, and high availability, including:
Healthcare, such as remote surgery and diagnosis of medical conditions;
- Entertainment and multimedia applications, such as 3D TV;
- Virtual reality, augmented reality and mixed reality, such as VR glasses;
- Tactile Internet of Things, providing ultra-responsive and ultra-reliable network connections, ensuring the delivery of control information and remote physical execution experience;
- URLLC to ensure reliable links between terminals;
- Internet of everything, such as smart appliances in smart homes;
- Smart factories, where employees remotely control machines to improve safety and productivity;
- Emergency response, making decisions based on collected data and information at critical moments;
· Intelligent transportation system, unmanned vehicles can perceive environmental information and make real-time responses autonomously; drivers can avoid dangerous road sections and reduce accidents by sharing or collecting information from traffic centers.
03 / Classification of edge computing
The classification of edge computing according to the target, computing platform, attribute, use of 5G functions, performance measurement and the role of edge computing in 5G is shown in Figure 2:
Figure 2 Classification of edge computing in 5G
A. Goal
The goals to be achieved by edge computing in 5G mainly include the following five types:
1. Improve data management, local real-time processing of a large amount of delay-sensitive data generated by the terminal;
2. Improve QoS to enhance user experience and help realize highly interactive applications and on-demand services;
3. Predicting network demand helps to decide whether to process locally at the edge or in the cloud, and provide optimal resource allocation;
4. Management location awareness enables the edge server to judge its own location and track the location of the terminal, realize location-based services, and enable location-based service providers to outsource services and data to the cloud;
5. Due to the limited resources available in the edge cloud, it is necessary to improve resource management, optimize the utilization of network resources, and improve network performance;
B. Computing platform
Different computing platforms have different computing power and characteristics (such as availability, network infrastructure complexity) in order to process different types of data. These platforms can be used alone or in combination according to specific needs and network scenarios. For example, for applications and services with strict QoS requirements, edge servers can be used to process real-time data, which is a case of use alone; while for healthcare applications and services that include both real-time and non-real-time data, edge servers can be used Processing real-time light-weight data and using the cloud to process heavy-weight data is a case of a combination of computing platforms. Computing platforms in 5G mainly include the following three:
1. Cloud computing: far away from the terminal is not suitable for real-time services;
2. Edge computing: close to the terminal, collect, process, and store data locally, and provide low-latency and delay analysis; Table 1 shows the comparison of the two edge computing platforms.
2.1 Fog computing: Deploy local fog nodes (local hardware devices) to provide local computing. It is a system-level horizontal architecture that distributes resources and services of computing, storage, control, and networking anywhere from the cloud to things; however, the storage capacity is low.
2.2 Multi-access edge computing MEC: Provide storage and computing capabilities at the edge of the network, such as BSs, to improve context awareness and reduce latency. MEC usually connects multiple hosts, and monitors hosts by collecting host information.
Table 1 Comparison between fog computing and MEC
3. Cloud computing and edge computing are used together to make non-real-time and real-time decisions respectively. Combining the advantages of both can greatly reduce delays, but the hybrid platform is more complicated.
c. attributes
Edge computing has three main attributes:
1. Low latency and close distance: the terminal is very close to the server, and the end-to-end delay and response delay are small.
2. Location-aware: Edge servers collect data from nearby sources without sending it to the cloud.
3. Aware of network context: adjust in real time to optimize resource utilization.
D. 5G functions related to edge computing
The five implementation factors of edge computing in 5G are:
1. Software-defined network SDN: Divide the network into control and data planes, simplifying network management and deploying new services.
2. Network function virtualization NFV: Network functions are executed in virtual machines, and network requirements are processed in the cloud or at the edge.
3. Massive MIMO: Increase antenna arrays to increase network capacity and energy efficiency; at the same time, multiple UEs can simultaneously offload tasks to edge servers to reduce delay and energy consumption.
4. Wireless access technology for dynamic access: Allows access to various devices, improving network scalability.
5. D2D communication: Adjacent UEs can communicate directly; edge computing endows UEs with computing capabilities and ensures the success of D2D.
E. The role of edge computing in 5G
1. Local storage: Data is offloaded from the UE to the edge cloud, and different storage strategies are provided for different types of data.
2. Local computing: The edge cloud provides terminal-like local computing and data processing capabilities in an independent manner.
3. Local data analysis: Perform data analysis directly locally, and then use the analysis results for decision-making.
4. Local decision-making: Edge computing helps entities make decisions, such as how to exercise control.
5. Local operation: Edge computing enables remote and monitoring, especially for critical equipment.
6. Local security enhancement: Due to the close proximity of edge computing, malicious entities can be quickly detected and isolated, and a response can be initiated.
F. Performance indicators
The performance of edge computing is brought about by its local functions:
1. Cost reduction: Through the local functions (R.1)-(R.5), it is no longer necessary to send to the cloud, reducing the consumption of network resources.
2. Higher QoS: Improve QoS and reduce delay through local functions.
3. Energy efficiency: Reduce energy consumption through local functions, that is, the energy required to offload tasks and data to the cloud, and improve energy efficiency.
04 / Current technology
A. Fog-based solutions
①Cross-layer resource management scheme: using a hybrid computing platform, the edge cloud performs real-time tasks, and the cloud server performs high-computation and resource-intensive tasks. Services for different applications are performed in three layers, namely cloud, fog and UE layers. Highly computing and resource-intensive services are executed in the centralized cloud layer, and the business is offloaded to the fog layer; the terminal layer performs functions locally on the terminal. The solution has the characteristics of low delay and proximity, and provides lower end-to-end delay (Qos).
② Energy efficiency model based on throughput and energy consumption: use fog computing to analyze fog computing and its energy consumption compared with cloud computing; use terminals to dynamically access rat and other 5G functions. This solution has the characteristics of low latency and proximity, and different rats (3G, 4G, 5G) can provide services to multiple different terminals.
B. MEC-based solutions
① Architecture for performing energy-aware offloading: Each mobile terminal decides whether to execute or offload computing tasks to the MEC server to reduce the energy consumption of the MEC.
The architecture optimizes data management and QoS through local computing and local decision-making; performs energy-aware functions through edge computing; and also uses 5G functions for dynamic access to rats. The three main steps of the scheme are as follows: First, classify according to the energy consumption of mobile terminals and the transmission delay between them and MEC (type 1 terminals use the MEC server to calculate, type 2 terminals can calculate by themselves, and type 3 terminals can choose to use MEC server or itself). Second, prioritize the terminals according to energy consumption, available channels, etc. (Type 1 terminals have higher priority and meet the delay constraints). Finally, assign channels with different priorities to the terminals.
② Prediction and active caching: Through local storage and local computing, the goal of improving data management and predicting network needs is achieved. Active caching is performed at the edge of the network or at the terminal using edge computing while utilizing 5G functions including D2D communication.
The solution has the characteristics of network context awareness, and can obtain network information and traffic distribution. During off-peak hours, frequently requested content is cached in edge servers, BSs or UEs. When a BS requests a specific content, there are two possibilities: a) the content has been processed or owned by an influential UE in the past, so it is sent from the UE to the BS via D2D; b) the content is not available on any influential terminal used, so it is sent from the core network to the BS. This solution can reduce operating costs.
C. Hybrid solution
① To enhance MEC, a D2D-based mobile edge and fog computing architecture is introduced to support collaborative computing to execute tasks in multiple computing platforms or paradigms. The architecture has properties of low latency and proximity. In this architecture, each terminal initiates a request and sends it to the nearest relay gateway, the relay gateway is connected to the core network or cloud necklace, and its service handler has information about the available services, it decides that the requested service is executed locally Or forward to another relay gateway that can perform the service. The decision is based on the availability of services at the relay gateway and its neighbors.
②Propose a real-time, context-aware, service composition and collaboration architecture to realize fast combined services, which is the amalgamation of multiple services supported by the collaboration of different hardware and software with different functions. The proposed architecture uses hybrid computing to support the collaboration among cloud, MEC and terminal. Frequently accessed blocks in this architecture are small units decomposed by files, which are stored or cached in the MEC server. The blocks requested by multiple servers will be copied and cached to other MEC servers according to the file type and content. This helps reduce the end-to-end latency associated with accessing the cloud.
05 / Unresolved issues
A. Improve service QoE
QoE is a measure of the customer's overall level of satisfaction with the service provider. It is related to, but different from, QoS. QoS embodies both hardware characteristics (such as memory quantity) and software characteristics (such as interface development). The current problem is how to achieve a balance between: a) higher availability or seamless linking of applications, which can be provided by the cloud when the terminal is away from the edge server; b) when the terminal is near the edge server, Edge cloud can provide higher application QoE to reduce latency and jitter.
B. Protocol standardization
Protocol standardization requires a set of generally accepted rules for edge computing in a 5G environment. Because the edge cloud is too flexible and the rules of different suppliers are customized, it is difficult to reach an agreement on a standard. At the same time, a large number of heterogeneous terminals use different interfaces when communicating with the edge cloud. These are the challenges in the protocol standardization process.
C. Heterogeneity issues
The heterogeneity of communication, computing and other technologies in edge computing in the 5G environment, such as the large differences in the data transmission range and transmission rate of different companies, makes it difficult to develop portable solutions across different environments. This is the heterogeneous problem.
D. Security and Privacy
In edge computing, data cannot be transmitted across the network, which enhances security and privacy to a certain extent, but there are still hidden dangers at the edge of the network. First, the dynamic environment will lead to rapid changes in the data and network requirements of different network entities; second, the increase in the number of intercommunicating devices requires a scalable solution. In order to increase the robustness of the network, these issues must be addressed, but may increase the complexity and cost.
06 / Summary
This paper reviews the latest developments in edge computing in 5G communications, including fog-based, MEC, and hybrid schemes, and introduces edge computing methods and their characteristics for different feature classifications. Key requirements for edge computing include real-time interaction, local processing, high speed, and high availability. The article points out the problems faced by 5G deployment of edge computing, such as service enhancement, standardization, heterogeneity, and security vulnerabilities, and briefly introduces the technology development direction. The deployment of edge computing in 5G has brought many benefits, but it has also brought some new problems, which require the joint efforts of researchers and society to solve.
references
[1] N. Hassan, K. -LA Yau and C. Wu, "Edge Computing in 5G: A Review," in IEEE Access, vol. 7, pp. 127276-127289, 2019, doi: 10.1109/ACCESS.2019.2938534 .
[2]1. WZ Khan E. Ahmed S. Hakak I. Yaqoob and A. Ahmed "Edge computing: A survey" Future Gener. Comput. Syst. vol. 97 pp. 219-235 Aug. 2019.
[3]K. Cao, Y. Liu, G. Meng and Q. Sun, "An Overview on Edge Computing Research," in IEEE Access, vol. 8, pp. 85714-85728, 2020, doi: 10.1109/ACCESS. 2020.2991734.