Let ChatGPT tell you how to build a lossless network that supports ChatGPT computing power
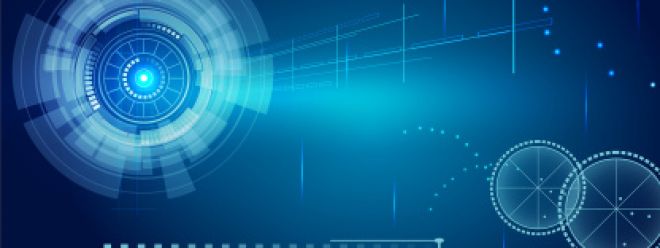
Let ChatGPT tell you how to build a lossless network that supports ChatGPT computing power
With the acceleration of the digital transformation of global enterprises, the current applications represented by ChatGPT continue to deepen in the fields of production and life. Behind the sudden increase in the popularity of ChatGPT, it represents the demand for infrastructure required by artificial intelligence automatic content generation technologies. .
In the next five years, the compound annual growth rate of my country's intelligent computing power scale will reach 52.3%
According to the "2022-2023 Evaluation Report on the Development of China's Artificial Intelligence Computing Power", the scale of China's intelligent computing power will reach 155.2 EFLOPS (FP16) in 2021, and it is expected that by 2026, the scale of China's intelligent computing power will reach 1271.4 EFLOPS. During the period from 2021 to 2026, the compound annual growth rate of China's intelligent computing power scale is expected to reach 52.3%.
With the introduction of national policy plans such as the "East Counting West" project and new infrastructure, my country's intelligent computing center has set off a construction boom. At present, more than 30 cities in my country are building or proposing to build smart computing centers. The overall layout is mainly in the eastern region and gradually expanding to the central and western regions. From the perspective of development foundation, around the development ideas of AI industrialization and industrial AI, the artificial intelligence industry has initially formed an architecture system center on heterogeneous chips, computing power facilities, algorithm models, and industrial applications. The intelligent computing center has a construction foundation.
Building a large-scale intelligent computing base
Currently ChatGPT's training model is mainly based on the general-purpose large model base GPT-3. Training super-large basic models requires various key technologies as support, and algorithms, computing power, and data are indispensable. Algorithms rely on the improvement of large model parameters and the optimization of the model itself, while computing power and data need to rely on traditional GPU servers, storage, and networks to achieve mutual promotion.
The data shows that the total computing power consumption of ChatGPT is about 3640PF-days (that is, if the calculation is 1 quadrillion times per second, it needs to be calculated for 3640 days), and it needs 7~8 data centers with an investment scale of 3 billion and a computing power of 500P to support the operation . According to the visit volume of 13 million/day, ChatGPT needs 30,000+ GPUs. During the training process, the GPU will communicate frequently, including P2P communication and Collective communication. Within a node, the communication interconnection bandwidth between GPUs can reach 400GB/s. Between nodes, GPU communication uses the RDMA network. With the support of GDR (GDR, GPU Direct RDMA) technology, the RDMA network card can bypass the CPU and memory, and directly read data from the remote node to the GPU memory.
At the network level of the computing power center, it is necessary to realize the integration and optimization of the network and application system through technologies such as intelligent lossless storage network, and to improve the overall throughput of the network and reduce network delay through flow control technology and congestion control technology.
For H3C's intelligent and lossless network , ultra-large-scale networking is the only way to build intelligent computing power. At present, AIGC represented by ChatGPT, including the significance of the large model behind it, is not only in the implementation itself, but its scientific research value may be even greater. It is generally believed that the first few industries to land may include scientific research, education, and Internet-related industries.
Taking the large-scale deployment of the Internet industry as an example, an Internet company followed chatGPT and other AI training as an opportunity to build a cluster computing network with a single PoD supporting 4,000 200G ports. The number of ports deployed in the current PoD in the intelligent computing center based on scientific research and education is usually between 1000 and 4000. Therefore, H3C provides a variety of optional high-performance network solutions to fully meet the scale of different business scenarios of users.
Box-box networking : the current main GPU server 100G/200G/400G network card rate, taking the latest S9825/S9855 series of three-layer ToR/Leaf/Spine networking architecture adopted by H3C as an example, Spine adopts dual planes and ensures ToR up and down The line convergence ratio meets the 1:1 requirement. When the server access rate is 400G, a single PoD can support 1024 servers, and the cluster can provide scale access of 2048 400G servers; if the rate of 200G is used, a single PoD can support 2048 servers, and the cluster can support a maximum of 32 PoDs, which can meet the theoretical requirements of 6.5 10,000 servers can be accessed on a scale; if 100G rate access is used, the cluster can be accessed with a maximum scale of more than 100,000 servers.
Figure 1: 200G access network with three-level box architecture
As for lossless networks with a deterministic scale, H3C provides a lightweight intelligent lossless network deployment solution of "one frame is lossless", which can also meet the intelligent computing networking needs of most scenarios.
Taking the S12516CR fully configured with 576 400G ports as an example, a single frame acts as a ToR and directly connects to the server network card to achieve 1:1 convergence. It can support a maximum of 576 400G QSFP DD ports on a single PoD; 200G QSFP56 can meet the maximum 1152 ports. input; and 100G QSFP56 can meet the maximum 1536 port access. It should be noted that more than 2,000 DR1-encapsulated 100G ports can be obtained by directly splitting 400G DR4, and the current mainstream network cards do not support DR1. The advantages of using a single-frame lossless architecture are obvious. Adopting a networking architecture that abandons the traditional Leaf/Spine architecture can effectively reduce the number of devices, reduce the number of data forwarding hops, and effectively reduce data forwarding delays. At the same time, there is no need to calculate the convergence ratio and equipment scale under multiple levels. etc., which greatly simplifies the difficulty of deployment and model selection and effectively improves the networking efficiency. It is a new attempt to intelligent lossless network of deterministic scale.
Figure 2: "One frame is lossless" 200G access networking
Box-box networking : For larger-scale networking needs, H3C data center network provides box-box lossless architecture.
Also taking the 100G/200G/400G network card rate of the GPU server as an example, if the S12500CR series, the flagship data center frame product of H3C, is used to construct the ToR/Leaf/Spine three-layer network architecture, a single S12516CR serves as the spine and ensures the ToR uplink and downlink convergence ratio Meet the 1:1 requirement. Ordering a PoD at a server access rate of 400G can support thousands of servers, and the cluster theory can provide a maximum scale access of nearly 59 400G servers; if the rate of 200G is used, a single PoD can support 2,000 servers, and the cluster can provide nearly 1.18 million servers Server-scale access; if a 100G rate is used to access the cluster, it can provide a maximum of over 2 million server-scale access. The figure below shows the 200G access network of the three-layer frame structure
Both large-scale networking and cell switching
For data center switches, whether it is a traditional frame or box switch, the port rate increases from 100G to 400G. Not only have to face the problem of power consumption, but also have to solve the Hash accuracy and elephant and mouse flow of box-type networking. Therefore, H3C data center switches give priority to adopting DDC (Distributed Disaggregated Chassis) technology to cope with the increasing computing power network solution when building an intelligent lossless computing power data center network. DDC technology distributes and decouples large chassis devices, uses box switches as forwarding line cards and switching fabric boards, and flexibly distributes them in multiple cabinets to optimize network scale and power consumption distribution. At the same time, DDC box switches Cell switching is still used between switches.
Names of roles in the DDC system:
NCP:Network Cloud Packet (Line card in Chassis)
NCF:Network Cloud Fabric (Fabric card in Chassis)
NCM: Network Cloud Management (Main Management card in Chassis)
Figure 4: DDC architecture
Figure 5: DDC architecture decoupling, 400G Full mesh full interconnection
Taking S12516CR as an example, a single device can support 2304 100G servers and support 1:1 convergence. The DDC solution decouples control terminals independently, adopts 400G full interconnection between NCP and NCF and supports cell forwarding at the same time, supports data center leaf and spine non-blocking, and effectively improves data packet forwarding efficiency. After testing, DDC has certain advantages in the Alltoall scenario, and the completion time is increased by 20-30%. At the same time, compared with traditional box-type networking, DDC hardware has obvious advantages in convergence performance. From the comparison of port up and down tests, it can be found that the convergence time of using DDC is less than 1% of the box-type networking time.
Network Intelligence + Traffic Visualization
The service mode of the intelligent computing center has changed from providing computing power to providing "algorithm + computing power". In the intelligent lossless network, the blessing of AI lossless algorithm is also needed.
The data traffic characteristics forwarded by each queue in the lossless network will change dynamically over time. When the network administrator statically sets the ECN threshold, it cannot meet the real-time dynamic changing network traffic characteristics. H3C's lossless network switches support AI ECN function to dynamically optimize the ECN threshold according to certain rules by using the AI service components locally on the device or on the analyzer. Among them, the AI business component is the key to realize ECN dynamic tuning, and it is a system process built into network devices or analyzers. It mainly includes three levels of functional frameworks:
- Data collection and analysis layer: Provides a data collection interface for obtaining a large amount of feature data to be analyzed, and preprocesses and analyzes the obtained data.
- Model management layer: manage model files, and obtain the AI ECN threshold based on the AI function model loaded by the user.
- Algorithm layer: Call the interface of the data acquisition and analysis layer to obtain real-time feature data, and calculate the AI ECN threshold according to the search trial algorithm with a fixed step.
Figure 6: Schematic diagram of AI ECN function realization
In addition, H3C data center network provides AI ECN operation and maintenance visualization. According to the different implementation positions of AI service components in the network, the AI ECN function can be divided into two modes: centralized AI ECN and distributed AI ECN:
- Distributed AI ECN: AI business components are integrated locally on the device, and a dedicated neural network (GPU) chip is added to the device to meet the computing power requirements of AI business components.
- Centralized AI ECN: AI business components are implemented by analyzers. Applicable to the future SDN network architecture, it facilitates centralized management, control and visual operation and maintenance of all AI services including AI ECN.
In the above two scenarios, the advantages of the SeerAnalyzer analyzer can be used to present the user with a visualized AI ECN parameter tuning effect.
Figure 7: PFC backpressure frame rate comparison before and after AI ECN tuning
Looking back on the past, H3C has reached in-depth cooperation with many leading companies in the field of intelligent and lossless networks. In the future, H3C's data center network will continue to focus on ultra-broadband, intelligent, integrated, and green evolution, and provide smarter, greener, and more powerful data center network products and solutions.
Citation:
1. Guangming.com: The popularity of ChatGPT drives the demand for computing power. Can the scale of computing power in my country support it?
2. "Guidelines for the Innovation and Development of Intelligent Computing Centers"
3. "DDC Technical White Paper"