Detailing the design points and principles of 6G system data governance scheme
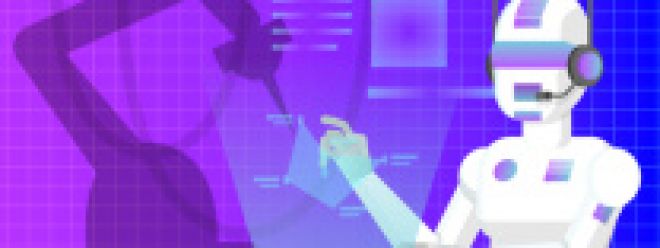
These data will also be exchanged with other systems and business areas as knowledge experience, generating broader value. This article is reprinted from WeChat public number "Big Data DT" by Tong Wen Zhu Peiying. To reprint this article, please contact Big Data DT Public. The scope of data use is different, and data governance itself is different in both economic and technical connotation. Data governance refers to the management, maintenance and deep development of data through relevant processes and technologies to obtain high-quality data that can be used as a key asset of an organization. Each Mobile Network Operator (MNO) segregates and stores data generated in mobile communication systems separately by technical domains, including Radio Access Network (RAN), Core Network (CN), Transport Network ( Transport Network (TN), and Operation, Administration, and Maintenance (OA&M). The data owned by different network elements and participants are not open and transparent enough, and the resulting data silos are the main bottleneck in data collection and sharing. On the other hand, large OTT (Over-The-Top) business companies have accumulated expertise in data governance and realization strategies (e.g. data storage, analytics services, API interfaces) far ahead of telecom sector companies. The data governance solution for 6G system will provide strong support for AI and sensing services, which will give rise to new business approaches and system features. I. Design points and principles The scope of data governance goes far beyond traditional data collection and storage. Overall, the system design needs to consider four aspects, as shown in Figure 1. ▲Figure 1 Design points of data governance 1. Data accessibility and quality Data accessibility and quality is one of the biggest challenges to the adoption of AI in various industries. Improving data accessibility means that data cannot just come from a single system and a single domain, but needs to come from different domains of multiple systems at the same time. This raises a fundamental question: how to break down the physical boundaries (between multiple vendors, operators, and industries) and get data into the heterogeneous data ocean? Once the otherwise scattered and isolated data is collected and utilized, another question arises: how to improve the quality of the data? The acquisition of massive amounts of data does not mean that the acquired data is usable and of high quality. At the same time, there is a need to improve the efficiency of data processing while considering reducing the computational complexity and energy consumption of data processing. 2. Data sovereignty With the all-digital transformation of society, the importance of data sovereignty, data security and privacy has never been more prominent, and many countries have enacted privacy protection laws and regulations. Service providers are also constantly updating their privacy protection schemes, and major national governments are developing or have issued regulations related to data management. For example, the EU's General Data Protection Regulation (GDPR), enacted in 2018, regulates the use of data at the EU level. in 2019, China enacted the Data Security Management Measures, which, together with the Cybersecurity Law enacted in 2016, constitute the Chinese version of the GDPR. The U.S. is also implementing privacy-related laws, such as California's Consumer Privacy Act (CPA), which went into effect in January 2020. The design of 6G systems should take into account regulatory uncertainties, especially those arising from regulatory differences between different regions. 3. Knowledge management Generally speaking, knowledge can be regarded as processed data with specific uses or values that can be directly used by physical or virtual entities in different technical and business domains. Knowledge management includes knowledge generation, updating and opening. For knowledge generation and updating, we need to carefully check the source and quality of data and take measures to intercept low-quality and harmful data generated by unreliable or even malicious data sources. And opening up knowledge as a capability to the public requires suitable platform and interface design. 4. Legal issues A wide variety of sensors and other technologies can generate data in real time, which makes data collection and use increasingly complex and sensitive. Increased data generation capabilities not only provide new data streams and content types, but also raise policy and legal concerns about data misuse: ulterior agencies or governments may use these capabilities for social control purposes. At the same time, new technological capabilities make it difficult for the average person to distinguish between the truth and falsity of technological content. For example, it is difficult for the average person to distinguish between a real video and a "deep fake" (deep fake) video. Protecting It is increasingly important to protect the fragile balance between protecting the social benefits of technology and preventing its ability to be used to enforce social control and deprive freedom. Stricter legal and policy instruments are needed to identify fraud and prevent the misuse of advanced technologies. II. Architectural Features Independent data facets are a key feature in the design of the data governance system (shown in Figure 2), which will provide data-related common capabilities for the 6G system, thus providing transparency, efficiency, endogenous security, and privacy protection for both internal and external functions of the 6G system. The basic concepts and related network functions and services are described in the following section. ▲ Figure 2 Independent Data Plane for Complete Data Governance 1. Independent Data Plane The independent data plane aims to implement a data governance scheme for 6G systems, which handles data from different business entities. Regardless of where the data comes from, the entire life cycle of data is processed in this plane, including data generation and collection, data processing and analysis, and data business issuance. As a result, a separate data plane can provide data services to external business entities (such as automotive, manufacturing, and healthcare verticals), as well as network automation and optimization services to the 6G system itself (such as the control plane, user plane, and management plane). Configuration, status, and logs related to network operation, as well as personal user data, sensor data, and data provided by other parties are all collected. The collected data results in a rich data resource that can be organized in a distributed form. To prevent problems caused by direct use of raw data for applications such as AI and perception, raw data usually requires pre-processing (e.g., anonymization, data reformatting, denoising, transformation, feature extraction, etc.) before it can be used. To ensure data integrity and process compliance, policies involved in data processing (e.g., regulations such as geographic restrictions, national or regional privacy regulations, etc.), whether or not they come from a regulatory level, need to be followed by default. The rights and obligations of data use agreed in the data contract also need to be complied with when passing data to the data surface. Data desensitization is key to protecting privacy, and the data surface needs to provide this service. All of the above services provided by the data facade are operated and managed by a self-contained OA&M system. Another important function of the data surface is to generate knowledge based on data collection, processing and organization. In order to coordinate the processing and transfer of data from different data sources, the production of knowledge also needs to be done according to contractual requirements. The data governance framework can evolve and be enriched as new data sources, data models, and data topics are noticed and used by data customers. Therefore, the operational management of the data governance framework and the real-time development of the framework can go hand in hand. Since data facets are a logical concept, they can be implemented through a centralized hierarchical architecture or as a logical function distributed over edge or deep edge nodes. Next we will explore some key elements of data facets. 2. Multiple Players of Data Governance The data governance ecosystem includes roles in two dimensions: from data customers to data providers, and from data owners to data managers. The different roles can be filled by different business entities. Therefore, data governance in 6G is a typical multi-party participation scenario, where data customers who use data or knowledge provided by 6G systems, and data providers of 6G systems may be involved. The 6G may have its own data governance framework, or it may build a data governance framework with other industry participants based on its own domain knowledge. That is, there may be different evolutionary or developmental routes for data governance frameworks. Therefore, it is important to determine how data rights are determined between different business entities in the operation phase, and this issue can be solved by decentralized technologies such as blockchain. 3. Data resources Data resources are very rich, including structured data, unstructured data, pre-processed data, post-processed data, and raw data. Efficient collection of data from wireless environments (e.g., user behavior data such as mobility and network state data) is a prerequisite for data governance. Intelligent methods can then be used to analyze the data and transfer data-derived knowledge to internal and external customers. It is therefore necessary to understand the sources of data. ▲Figure 3 Main data source categories Figure 3 illustrates some of the major data source categories in a 6G system. Infrastructure: The infrastructure, i.e., the communication system, includes various types of physical and virtual resources such as RAN, TN, and CN, as well as computing resources such as cloud, edge, and deep edge. The data generated within the infrastructure includes information about computing resources, information about communication resources (e.g., the state of a particular network function), sensory information (e.g., sensory information from the RAN), and certain user information (e.g., mobility information, location, and associated context). Operation Support System (OSS): The data in this layer includes all OA&M related data, such as physical equipment status, system operation information, and service issuance information. Business Support System (BSS): The data in this layer includes all the data related to business logic, such as customer information and partnership management information. What's more important is also the subscription data of consumers and enterprise customers, for which they should have full ownership and control. Industry communication systems: Data collected in 6G industry application scenarios may also include industry-related OA&M data information, industry subscriber information (such as traffic patterns and mobility data), and business/service data stored in the cloud. The ownership of such data should belong entirely to the industry customer. Terminal: Data from the terminal side includes computing and communication resources, service usage profiles, perceptual knowledge, etc. The ownership of such data shall fully belong to the end-user. 4. Data Collection In 6G, one of the main roles of data governance is to provide a suitable method to build data resources, which requires the support of suitable architecture and network functions. The first step to build data resources is to collect data, and there are several key actions in this step as follows. Establishing agreements (e.g., data authorization) and secure connections with data sources. Receive data collection requirements, determine the scope of collection, and determine where, when, and how to collect based on the requirements. Inform the data source of the data attributes. Collects data from data sources and accessions them. Operate and maintain the data in the database. 5. Data Analysis Based on the management of data resources, it becomes possible to provide data analysis services for different types of customers. There are four types of data analysis services that can be provided as follows. Descriptive analysis mines the statistical information of historical data to provide network insight information, such as network performance, traffic model, channel status, users, and other aspects. Diagnostic analysis enables autonomous detection of network failures and service impairments, and identifies the root cause of network anomalies, thereby improving network reliability and security. Predictive analytics uses data to predict future events such as traffic patterns, user locations, user behavior and preferences, resource availability, and even failures. Suggestive analytics provides recommendations for resource allocation, content presentation, etc. based on predictive analytics. Data facets provide knowledge from data analytics services that provide both active knowledge (e.g., action recommendations) and passive knowledge (e.g., information sharing and customers' action decisions). Data analytics services can be based on customer needs and customized to meet customer requirements. The data surface should be open to services and data in multiple dimensions on demand, and Table 1 gives examples of the types of services that can be offered to customers. As can be expected, the actual types of customers are richer than those listed in the table, and customers have different needs and usage scenarios for data analytics. Table 1 Examples of multidimensional data services offered by Data Surface 6. Data Desensitization Collecting and storing sensitive data involves privacy risks and requires privacy protection responsibilities. Data desensitization is an important action to respond to privacy concerns and achieve legal compliance, and is also particularly important for supporting AI and awareness services in 6G designs. For AI tasks in particular, cross-domain designs need to be considered. There has been a significant amount of recent research on differential privacy in AI, exploring how to anonymize training data from individual devices. Data desensitization during model training and AI inference is essential in 6G design. Methods to achieve differential privacy include: adding noise to the training data without affecting the statistical properties of the data, where the training model can still capture the features of the original dataset; and using encryption techniques so that machine learning is performed based on encrypted (rather than decrypted) data. There is also an approach that allows the device to send model parameters instead of training data, such as federation learning and split learning. There is a risk in this process that if an insider with full control of the learning method has bad intentions, then he can use the gradual convergence of the model to construct information similar to the training data. In federation learning, for example, the information could thus be leaked to a malicious device. Regardless of the learning method, data desensitization is an issue that needs to be considered. Therefore, we need to think about how to deal with the differences between different learning methods and the limitations of the learning methods themselves under this premise. About the author: Dr. Wen Tong, CTO of Huawei Wireless, Chief Scientist of Huawei 5G, Fellow of Huawei, IEEE Fellow, Member of the Canadian Academy of Engineering, recipient of IEEE Communications Society Outstanding Industry Leader Award and Fessenden Medal. Dr. Peiying Zhu, Senior Vice President of Wireless Research Area, Huawei, Fellow of Huawei, IEEE Fellow, and Member of the Canadian Academy of Engineering.