Is your network AI as smart as you think?
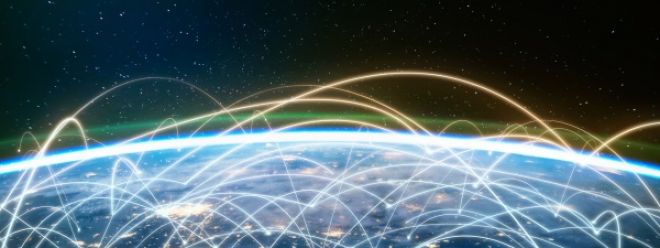
Network operators told me that in the future, artificial intelligence
will manage their networks. They also told me that the supplier had
communicated the same message to them. The good news is that "some
aspects" are true. The bad news is the same.
Network operators told me that in the future, artificial intelligence
will manage their networks. They also told me that the supplier had
communicated the same message to them. The good news is that "some
aspects" are true. The bad news is the same. The focus here is on
"some aspects." In order to get the most benefit from artificial
intelligence network management, you must figure out the vague "some
aspects". For this, you can realize this by imagining "ants and
farmers".
Ants can build extremely complex anthills with various interconnected
tunnels and checkpoints. Do these worker ants have some powerful ant engineers
to guide the completion of this process? The answer is no! They each perform
their own simple tasks wholeheartedly and complete tasks instinctively. In
fact, there is an "ant engineer", but it is their own DNA that
organizes their work to achieve their goals. This is a bit like the way most
network AIs work.
The network is composed of a bunch of technology
"collections", each of which is a bit like an anthill. There are
collections based on supplier, device type, physical location, and connection
relationship. If you look closely at today's network AI, you will find that it
mainly runs on "collections." Maybe it manages Wi-Fi or it may manage
edge elements such as SD-WAN or SASE. AI applications used to manage a
"collection" will incorporate management goals into their DNA (that
is, their design). Simply put, if we are a Wi-Fi supplier, we know how Wi-Fi
works and incorporate this knowledge into our AI management.
The challenge comes when we no longer think of collections as independent elements and start to think of networks as "collections of collections". The network is not an anthill, but the entire ecosystem where the anthill is located, including trees, cows, and many other things. The tree knows how to become a tree, and the cow understands the habits of the cow, but who understands the entire ecosystem? A farm is a farm, not any combination of trees, cows and anthills. The person who understands what a farm should be is the farmer, not the elements of the farm or the suppliers of these elements, in your network, dear network operators, the farmer is you!
In the early stages, the developers of artificial intelligence clearly
admitted that the knowledge engineers who build the AI framework are separate from the subject matter experts (whose
knowledge shapes the framework). In software, especially DevOps, management
tools are designed to achieve a target state, that is, in our farm analogy, it
describes the location of cows, trees, and ants. If the current state is not
the target state, they will do something or move something to move closer to
the target state. This is a great concept, but to make it work, we must know
what the goal is. At the corporate network level, we need our Wi-Fi experts to
subconsciously introduce knowledge into Wi-Fi AI management tools. If AI
vendors don’t know how this knowledge was acquired, their AI will be useless.
If your hopes for AI are dashed, it is still too early to say! Many
network operators are still very satisfied with the AI that manages the technology collection that makes up their network.
After all, when nothing happens to one set can be remedied by adjusting the
other, why worry about coordinating Wi-Fi and SD-WAN management? If this set-AI
model can meet your needs, you're done NS.
To understand whether it is feasible to be an "ant" (at
least in terms of network AI), the best way is to ask whether your technology
collection is really "atomic"—that is, completely independent and
self-contained. This boils down to the visibility and control range of your AI.
Basically, the set-specific AIs are all independent. Ideally, you need your AI
to gather ants to do their own things instead of intervening in each other's
activities. You don't want the AI in one place to view another set without coordination and react to
conditions, or the two AI set processes deal with the same problem at the same
time.
If the remedy for a problem in one set might involve doing something
to another set, then you need your AI to be able to cover the combination.
Therefore, if you feel that the network operations center used to manage the
ecosystem is too expensive and overloaded, and want to deploy AI so that
everyone can relax, then you need to understand the supplier's AI proposition
more deeply.
The challenge here is to find the target state and how to recover when
something goes wrong. Remember the "subject experts" and
"knowledge engineers" mentioned above? It is difficult for us to
build AI solutions for networks because all networks are slightly different,
and only users themselves know what they think is "good" or
"bad" . Some AI tools may provide machine learning (ML) capabilities
to let your network operations center (NOC) personnel understand the situation
and know what to do; others may use what the network provider knows usually
represents normal options and common remedies Baseline.
However, both of these methods have some problems. Machine learning
may take time, and when your AI system is performing its mission, it will
further consume your NOC resources. When your network is mainly composed of
equipment from one supplier, the supplier baseline is most effective. Both can
be adjusted, but both may conflict with adaptive network behavior.
IP networks essentially use topology to discover and do their own
thing. Even for NOCs, it is difficult to influence routing; they usually need
to plan new MPLS routes for traffic engineering, and AI is unlikely to do this.
Some companies (including Google) have turned to software-defined networking
(SDN) to provide central control of routing, and AI can then control the
network by controlling the SDN controller.
AI in network operations can be traced back to a combination of events
to send out abnormal signals, and it can also be used as a way to implement
effective responses. At any level, your potential AI supplier should be able to
discuss with you how their products collect information and how to realize
their insights. Delve into the details of these two things, because no matter
how magical AI claims, it won't work without these two ingredients. Finally,
please remember: to be a farmer, not an ant, your position will never be lost
due to the emergence and development of AI.