AI Data Cycle: The Best Storage Combination for Large-Scale AI Workloads
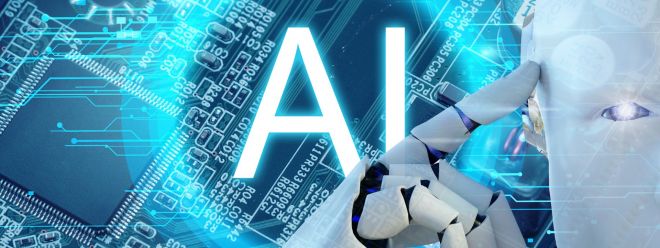
Although AI is revolutionizing people's lives and inspiring a variety of new applications , fundamentally , its core is data usage and data generation.
As the AI industry builds out vast new infrastructure to train AI models and deliver AI services ( inference ) , there are important implications for data storage. First, storage technology plays a major role in the cost and energy efficiency of each stage of this new infrastructure. As AI systems process and analyze existing data, new data is generated , much of which will be stored because it is useful . New AI use cases and more complex models make existing repositories and additional data sources more valuable for model context and training, driving the cycle: increased data generation drives data storage expansion , which drives further data generation , a virtuous AI data cycle.
For enterprise data center planners, it is important to understand the dynamic relationship between AI and data storage . The AI Data Cycle outlines storage priorities for large-scale AI workloads in each of the six phases . Storage component manufacturers are adjusting their product roadmaps, recognizing these accelerated AI-driven demands : maximize performance and, at the latest, reduce total cost of ownership (CTO) .
Let’s take a quick look at the stages of the AI data cycle :
Raw data archiving and content storage
Collect and store raw data securely and efficiently from a variety of sources . The quality and diversity of the data collected is critical, as it lays the foundation for everything that follows.
Storage needs : High-capacity enterprise hard disk drives (eHDDs) remain the technology of choice for low-cost bulk data storage, continuing to offer the highest capacity per drive and the lowest cost per bit.
Data preparation and ingestion
Data is processed, cleaned, and transformed as input for model training. Data center owners are implementing upgraded storage infrastructure , such as fast data lakes , to support data preparation and ingestion.
Storage needs : All-flash storage systems incorporating large- capacity enterprise solid-state drives (eSSDs) are being deployed to augment existing HDD- based repositories or in new all-flash storage tiers.
AI model training
It is at this stage that the AI model is iteratively trained to make accurate predictions based on the training data. Specifically, the model is trained on high-performance supercomputers, and the training efficiency depends largely on maximizing GPU utilization.
Storage requirements : Ultra-high- bandwidth flash storage close to the training servers is important to ensure maximum utilization. High-performance ( PCIe® Gen. 5 ) and eSSDs optimized for low-latency computing are designed to meet these stringent requirements.
Reasoning and Hints
This phase creates user-friendly interfaces for AI models, including APIs , dashboards, and tools that combine context- specific data with end-user prompts. AI models are integrated into existing Internet and client applications, enhancing them without replacing existing systems. This means maintaining current systems as well as new AI calculations, driving further storage requirements.
Storage needs : Current storage systems will be upgraded to accommodate additional data center eHDD and eSSD capacity to integrate AI into existing processes. Similarly, to enhance existing application systems with AI, larger capacity , higher performance client SSDs (cSSDs) for PCs and laptops and larger capacity embedded flash devices for mobile phones, IoT systems, and automobiles will be needed .
AI Inference Engine
The fifth stage is where the magic happens in real time. This stage involves deploying the trained model into a production environment where the model can analyze new data and provide real-time predictions or generate new content. The efficiency of the inference engine is critical to timely and accurate AI responses.
Storage requirements : High-capacity eSSDs for streaming context or model data to inference servers ; high-performance computing eSSDs for caching , depending on scale or response time goals ; high-capacity cSSDs and larger embedded flash modules in AI - enabled edge devices .
New content generation
The final stage is the creation of new content. The insights gained by AI models often generate new data, which is stored because they have proven to be valuable or interesting. While closing the loop at this stage, it also feeds back into the data cycle, driving continuous improvement and innovation by increasing the value of training data or for future model analysis .
Storage needs : Generated content will be returned to high-capacity enterprise eHDDs for archival data center storage , as well as high-capacity cSSDs and embedded flash devices in AI -enabled edge devices .
A self-perpetuating cycle of increased data generation
This continuous cycle of data generation and consumption is accelerating the need for performance-driven , scalable storage technologies to manage large AI datasets and efficiently restructure complex data to drive further innovation.
IDC Research Director Ed Burns noted : “ Storage is expected to have a significant impact as the role of storage and data access impacts the speed, efficiency, and accuracy of AI models , especially as larger , higher -quality datasets become more common . ”
There is no doubt that AI is the transformative technology. As AI technology is integrated into almost all industry sectors , storage component suppliers are expected to increasingly tailor their products to the needs of each stage in the cycle .
Original title: The AI Data Cycle: Understanding the Optimal Storage Mix for AI Workloads at Scale, author: Dan Steere