Is GPU the only lifeline for building large models? Not necessarily
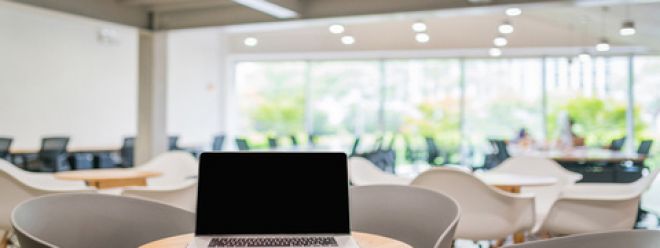
Ilya, former chief scientist of OpenAI, once publicly stated: “GPU is the Bitcoin of the new era.”
As large models take off, the demand for computing power surges. As the "shovel seller" in the AI gold rush, Nvidia has become the biggest winner in this round of technological change. As the AI arms race escalates, even though GPU prices are rising, it is often "hard to find a card" on the market.
On the one hand, GPU production capacity is tight and cannot keep up with demand; on the other hand, if the computing power supply is controlled by others, it will be equivalent to handing over the leading window period to others. In this context, many manufacturers either develop their own chips or look for alternative solutions and find new computing power solutions besides GPUs.
So, facing the dilemma of "hard to get a card", how to break the dilemma? What is the mystery behind Apple's abandonment of GPU and choice of TPU? How can domestic chip startups break through the track monopolized by giants?
This issue of "AIGC Practitioner" invited Yang Gongyifan, founder and CEO of Zhonghao Xinying, and Cai Zhewen, partner of SAI Zhibo Investment, to discuss the above topics.
1. GPU will not be the end of the entire AI model
In the current AI chip market, NVIDIA is the only one that stands out. In Cai Zhewen's opinion, the reason why NVIDIA can occupy the current ecological niche is that "30% is determined by fate and 70% is determined by hard work."
He said that Nvidia's success is first of all due to its grasp of the general trend of AI technology development. As the saying goes, "Heroes are made by the times", with the rise of large models, generative AI has blossomed everywhere, and the demand for computing power has surged. It just so happens that there is a lack of chips specifically for this field in the market. Nvidia's GPU has become a natural and suitable choice, thus occupying the market first.
More importantly, NVIDIA has made unremitting efforts in the process of development. "Around 2006, NVIDIA launched the CUDA system. Initially, it faced great internal resistance. After all, it was not something that could make money. But in the end, NVIDIA persisted and insisted on promoting this system, so that everyone would accept and recognize the ecosystem built around it, and naturally use its chips." In the end, NVIDIA successfully cultivated user habits, established brand loyalty, and created continuous demand for its products.
So will Nvidia continue to lead the pack? Not necessarily.
"From a product and technology perspective, we don't think NVIDIA's GPU will be the end point of the entire AI big model." Yang Gongyifan made such a judgment.
The young founder, who chose to return to China to start a business at a critical juncture in his life, pointed out directly: "Because this market is too large, it will cause people to have 'misunderstandings' about many phenomena in the market. Why can NVIDIA GPU form a 'monopoly' now? Because professional chips have not yet been released. Professional chips are still on the road to design and mass production, but at this time the entire industry application has exploded."
Throughout human history, the entire development process of semiconductors has always undergone major changes in a decade-long cycle. Each change is because the demand for existing applications exceeds the capabilities of existing tools. When this critical point arrives, new technologies and products will naturally emerge.
Yang Gongyifan said: The current explosion of AI is at such a node. Although various applications can use existing chips to meet the needs in the early stage, with the deepening of applications and the expansion of the market, the emergence of professional AI chips will inevitably change the market landscape.
"(In the future) GPUs may only occupy 10% to 20% of the market, and the remaining 80% of the market will be occupied by new AI chips. We hope that TPU will become the main force in the 80% market share. This is our vision and the reason why we established Zhonghao Xinying in China."
2. Challenging Nvidia: Looking for a way out
Of course, some people say that Nvidia’s graphics cards may not be the most suitable AI training tools, but its CUDA ecosystem is the only one in the world.
Due to the popularity of CUDA, a large number of developers and researchers began to develop applications based on CUDA, forming a huge user base and application ecosystem. This broad application base has created a strong ecological barrier for NVIDIA GPUs, making it difficult for other competitors to reach. However, with the development of technology and changes in market demand, the limitations of CUDA have gradually been exposed. Some startups and teams are trying to get rid of CUDA and seek to develop more efficient solutions that are more adapted to specific needs.
Yang Gongyifan believes that any industry, including the artificial intelligence industry, can generally be divided into two stages: in the R&D stage, iteration speed is the key, so developers tend to use more familiar tools, and whether these tools are the best cost-effective is not the main consideration; in the productization and commercial operation stage, especially large-scale deployment often leads to increased cost sensitivity, at this time, cost-effectiveness often becomes a key factor. This is why, although the CUDA ecosystem is mature, its disadvantages in cost-effectiveness will be revealed in the industrialization stage.
"Because all general-purpose things come at the cost of absolute performance loss." Yang Gongyifan emphasized that although CUDA, as a general-purpose software stack, provides broad support, this generality comes at the cost of sacrificing certain performance. In specific application scenarios, this performance loss may lead to a low cost-effectiveness, which in turn prompts the industry to seek more customized and optimized software stacks.
Another point worth noting is that NVIDIA is not only a GPU manufacturer, but also an important builder of large models. Unfortunately, despite NVIDIA's huge investment in the field of large models, its GPU architecture and CUDA software stack may not meet the higher requirements of computing performance, cost-effectiveness and network interconnection in future technological evolution.
Yang Gongyifan pointed out that for a technology company, especially a chip company, "it has no possibility to change its core architecture and completely revolutionize itself". Because this involves redesigning and developing from scratch, which is a long and complicated process. Correspondingly, the software stack built on it also has to start from scratch. In other words, whether it is a chip or a software stack, subsequent iterations are based on the experimental results and real scenarios of the previous generation of products.
To some extent, "the biggest advantage of GPU may be CUDA, but its biggest disadvantage is also CUDA."
"Because the CUDA software stack limits it. If I decide not to use GPUs in the future and instead adopt other hardware architectures that are more suitable for deep learning tasks, such as TPUs and LPUs, this inertial dependence will become its limiting condition. Although GPUs can improve performance through optimization, there is a theoretical ceiling. In contrast, chips designed specifically for AI, such as TPUs, may have a much higher performance ceiling than GPUs. As the application of large models is implemented and industrialized on a large scale, more effective AI chips such as TPUs may usher in an explosion because they can provide higher performance and lower costs."
Cai Zhewen also agreed with this. In his opinion, GPU will become less relevant one day, just as GPU replaced the CPU in graphics processing. Now there are chips designed specifically for AI, which are more efficient than GPU in processing AI tasks. As long as the entire AI application scenario continues to iterate in the future and the entire market becomes large enough, it is an inevitable trend that dedicated chips will gradually replace GPU in the field of AI.
In addition, Cai Zhewen also mentioned that although GPUs excel in parallel processing, their energy consumption is relatively high. With the increase in energy efficiency requirements, high energy consumption may become a disadvantage of GPUs in the field of AI, especially in large-scale computing tasks. Differences in power supply and new energy technologies in different regions may affect the choice of AI hardware. If the high energy consumption of GPUs becomes a limiting factor, and dedicated AI chips can provide lower energy consumption and higher performance, they may become a more popular choice.
3. TPU Revelation: Google's Past & Apple's Choice
As the wheel of history rolls forward, GPU may no longer have the status it has today, but GPU still dominates the hardware supply in the current AI era. Even in such a strong environment, Google TPU has still passed many tests and grown into a truly competitive opponent through the test of time.
In May 2016, Google first announced TPU at the I/O conference and said that the chip had been used in Google data centers for a year. When Lee Sedol played against AlphaGo, Google directly called TPU the "secret weapon" that AlphaGo used to defeat Lee Sedol. So why did Google insist on developing TPU when it already had GPU?
Yang Gongyifan mentioned that the process of Google developing TPU is actually a story of "an unexpected success". The creation of TPU is not the result of direct planning by Google's top management, but a process of spontaneous exploration and gradual verification by the internal team, and then seizing the opportunity of the times to realize commercialization.
Initially, it was born from an internal entrepreneurial project. Because the entrepreneurial environment within Google allows teams to conduct independent exploration and innovation, TPU is the product of this mechanism. However, given that the growth potential and monetization speed of software projects are much greater than hardware, the value of TPU itself does not meet the vision of the founder, and it needs to verify its development potential in specific fields.
As a result, TPUs were circulated among different departments within Google and tested in different application scenarios. Fortunately, through continuous trials and iterations, TPUs gradually demonstrated their efficiency and cost advantages in model training and reasoning. In particular, after being used by Google's advertising department, the accuracy of the recommendation system has improved, which is directly related to revenue growth, proving the commercial value of TPUs.
This also provided motivation for Google to continue to invest resources in the research and development and iteration of TPU. Ultimately, with the development of AI technology and the rise of large models, TPU has become an important competitive advantage for Google in the field of AI.
However, for a long time, TPU was still developing in the shadow of GPU. Until recently, when Apple announced the details of Apple Intelligence, TPU was once again put in the spotlight. According to the relevant papers, Apple did not use the common NVIDIA H100 GPU, but chose Google's TPU to train the basic model of Apple Intelligence, which caused a lot of discussion.
In this regard, Yang Gongyifan said that at first TPU was a technology used by Google itself and was not open to external use, but its open source culture indicates that it will eventually open TPU clusters as part of cloud services to promote the development of the entire industry. Apple is the first large player besides Google to use TPU for large model training.
"From a technical perspective, its main commercial driver is still cost-effectiveness." Yang Gongyifan introduced that under the same process, technology and energy consumption conditions, due to the particularity of its architecture, TPU has a higher chip utilization rate in the field of deep learning and large models, which can usually achieve a 3 to 5 times performance improvement, and the cost can be reduced by 50% at the same computing power. In commercial applications, cost savings become crucial, and the high cost-effectiveness of TPU becomes a key advantage. Therefore, as the industry develops, dedicated chips like TPU are likely to become the mainstream computing power platform.
Cai Zhewen analyzed Apple's choice from an industry perspective. In his opinion, there are four main reasons why Apple turned to TPU:
First, it is market-driven. With the development of artificial intelligence, the market needs more cost-effective and easy-to-copy technologies. Second, it is technological evolution. Initially, artificial intelligence lacked dedicated chips, and GPUs were widely used as a stopgap measure. But now with the surge in demand, more cost-effective chips are needed. Third, the law of market competition. Nvidia currently occupies a dominant position, but this has also inspired competitors to develop new chips optimized for AI to challenge its position. Especially for small and medium-sized emerging companies, it is a good opportunity to get involved in the TPU field. Fourth, it is a natural match. TPU originated from Google and has a natural advantage in compatibility and commercial matching with Google's large model framework. Overall, Apple's choice is both accidental and inevitable.