How to Choose a Large Language Model That Suits Enterprise Needs
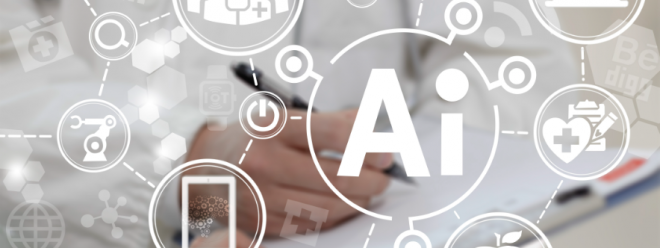
LLM is driving breakthroughs and efficiencies across industries. When choosing a model, companies should consider expected adoption, speed, security, cost, language, and ease of use.
Although generative AI is relatively new compared to other artificial intelligence (AI) technologies, it is already being used to support a range of tasks from reviewing job applicants to diagnosing and recommending disease treatments. IDC predicts that by 2028, 80% of CIOs will rely on generative AI tools to speed up analysis, facilitate decision-making, and improve customer service.
As companies race to tap into the technology’s potential to improve customer satisfaction and employee productivity, they are considering using large language models (LLMs) that are best suited to support generative AI applications, such as AI copilots and chatbots.
Learn about the diversity of LLM
The large number of LLMs available today means that companies are more likely to find an LLM that fits their specific needs rather than resorting to a one-size-fits-all LLM. This can speed up innovation, but choosing the right model from among the hundreds can be complex.
When choosing an LLM, companies should consider their expected applications, speed, security, cost, language, and ease of use.
Model types include:
- Business Model: Popular in the healthcare and financial services industries, this type of model is often used for projects that involve specialized customization or security constraints.
- Open source models: These models are often used by startups and small businesses for research due to accessibility and cost advantages.
- General purpose models: These models are trained using large amounts of data and can be used as a base model for building custom AI applications.
- Domain-specific models: These models are trained to fit a specific industry or use case, such as healthcare or financial services.
- Task-specific models: These tailor-made models are optimized for a single natural language processing (NLP) function, such as summarization, question answering, or translation.
- Visual Language Models: These models, called VLMs, combine computer vision and NLP to generate images from text descriptions and recognize objects from images. Using both text and code allows them to create and understand images without being trained directly on visual data.
It is also important to consider the size of the model as this will affect its capabilities and limitations. Some factors include the following:
- Inference speed: Smaller models generally provide shorter inference times, enabling real-time processing, improving energy efficiency, and saving costs.
- Accuracy: Larger models improved with Retrieval-Augmented Generation (RAG) often achieve higher accuracy.
- Deployability: Smaller models are well suited for edge devices and mobile applications, while larger models are best run in the cloud or in a data center.
- Cost: Larger models require more computing infrastructure to run.
Developers should also consider which languages the AI model must support based on who will use it and where it will be used. This is particularly important in the modern workplace, where employees may speak multiple different languages. Ensuring that the model can seamlessly translate languages is critical for effective communication and collaboration between users.
In addition, as sovereign AI becomes increasingly important, many countries are building proprietary models trained using local languages and data sets. This enables countries to maintain control and autonomy over AI, ensuring that the development and application of these technologies are consistent with their unique cultural, ethical, and legal standards.
How do businesses use LLM?
LLM supports a variety of AI applications, including chatbots and predictive analytics tools, which are bringing breakthroughs and efficiencies across industries.
- Healthcare: Insilico Medicine, a generative AI-based drug discovery company, has developed a new LLM transformer, nach0, to answer biomedical questions and synthesize new molecules. This multi-domain model enables researchers to efficiently process and analyze large data sets, reducing memory requirements, increasing processing speed, and facilitating more efficient data management and organization.
- Telecommunications: Amdocs is using its amAIz platform to improve business efficiency, drive new revenue streams, and improve the customer experience. This includes a customer billing agent that enables immediate access to LLM-based data insights and automation to resolve customer billing issues.
- Financial Services: Bank Negara (also known as BNI) is integrating Cloudera’s AI Inference service to enhance customer experience using generative AI and improve operational efficiency. This will enable BNI to efficiently deploy and manage large-scale AI models in a secure enterprise environment with high performance and data privacy.
Different models tailored to specific needs allow for the rapid implementation of AI solutions and tools to help automate redundant work. This frees up more time and space for people to focus on projects that bring value to the company and organization.
Looking ahead, developers will strive to build and deploy LLMs that enhance industry-specific applications, working to improve interoperability between systems, reduce operating costs, and increase efficiency. Using tailored LLMs, companies can build AI applications that meet their unique needs to improve customer satisfaction and promote operational excellence.