Let’s Talk About Data Analysis Models: People-Goods-Field Model
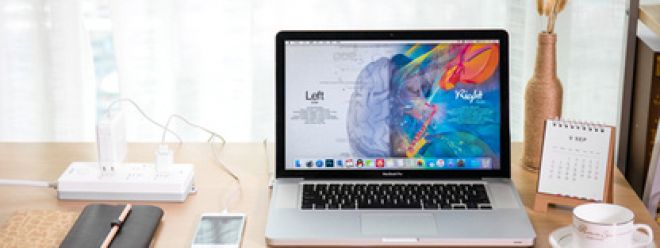
"I see that revenue is down/new users are down/costs are up, how can I analyze it further?" Many people have similar confusions, and often write a lot of year-on-year and month-on-month data, but don't know what to write except "year-on-year decrease, we need to make it higher." What should I do?
Here is a simple, practical, and easy-to-use analysis model that can solve 80% of common problems. This is the "people, goods, and venue" model. It is not an algorithmic model, so you don't need to understand complex statistical principles. You can use it as long as you are familiar with the business.
What is the people-cargo-field model?
The people-goods-field model is a fixed analysis routine. When we analyze a problem, we go deeper into these three dimensions to get the answer:
- People: Customers, Salesperson
- Goods: Commodity structure, commodity price
- Field: sales channels, promotion methods
This operation seems simple, but it solves a big pain point, which is the problem of "how to implement data analysis?"
- All they say is: "Income is too low, we need to increase it," but no one knows how to do it.
- You said: "The income is low because other people don't know Xiao Zhang's method", the business owner immediately understood it and went back to learn it!
This is how we go from data to business action. So, how do we do it?
How to apply the people-cargo-yard model
Step 1: Business sorting, first understand the basic data.
1. Who are our customers?
2. Who are the sales people in our company?
3. What products (goods) does our company have?
4. What channels (venues) does our company have?
The second step is labeling and classification. This step is crucial because the database may only contain specific customer names/specific product codes/channel codes. It is impossible to analyze these trivial and abstract things, so general classification is needed.
Common categories, such as:
1. C-end customers (people) are classified according to their cumulative consumption/membership level to distinguish between high and low purchasing power customers;
2. B-side customers (people), classified by industry/company size (large companies naturally have greater demand, so we should strive for them)
3. Salespersons (people), classified by work experience, past performance level, and number of old customers on hand
4. Commodities (goods), according to the natural attributes of the goods, make first, second and third level classification + commodity gross profit
5. Offline channels: classified by region, location, business area, and operating costs
6. Online channels: classified by promotion platform, cost, and conversion rate
After this step, a classified mind map needs to be formed to facilitate the formation of ideas during analysis; at the same time, labels should be added in the database to facilitate cross-comparison later (as shown below).
Step 3: Clarify the analysis goal. This is necessary for all analyses, and the goal should be specific:
- Wrong goal: Sales have dropped, analyze it
- Correct target: Sales in September only reached 85.3% of the target. Analyze the source of the difference
The analysis target can also be the number of new registered users/operating unit cost/product inventory, etc., as long as it is specific.
Step 4: Disassemble from the perspective of people, goods, and venue to find the cause of the problem. Here are some points to note:
1. Look at the big categories first, then the small categories
2. Start with the dimensions with the largest differences
3. Read one category first, then read another
For example, "Sales in September only reached 85.3% of the target." You can first see which of the following dimensions has the lowest target rate and where to start:
1. Compliance rate of different sales channels
2. Compliance rate of different product categories
3. Target achievement rate for different customers
Here you need to adjust the entry dimension according to the data situation. For example, in the figure below, although it seems that both teams have not achieved the goal (people), from the perspective of products (goods), the problem is actually caused by the new products not meeting the standards. At this time, don’t worry about the problems of teams AB, but switch the new and old products as the main analysis dimension and continue to dig deeper into the reasons.
After this step of disassembly, the main problem points can be identified and then in-depth analysis can be performed.
Step 5: Combine process indicators and conduct in-depth analysis of the reasons. For example, in the above example, we found that the new product's compliance with the standards was very poor. To explain why it was poor, more detailed data is needed, such as:
1. Comparison of basic product performance parameters with competing products
2. Comparison of product prices with competing products
3. Comparison of product promotion investment with similar products during the same period
4. Product distribution progress, comparison between plan and actual execution
This will allow you to see exactly where the problem lies.
This step is crucial for data implementation. However, some companies do not collect complete data, resulting in a lot of missing process data. The only way to make up for this is to bring the business together for a meeting, confirm the details, and then observe the rectification effect.
People-goods-yard models for different industries
Different industries have differences when constructing the people-goods-yard model:
1. For those with physical stores, the store location is particularly important! Therefore, label and classify the stores first.
2. For business, large customer orders are very important, so we prioritize classifying the customer industry/scale
3. For Internet products, there are a lot of C-end users, so we first stratify users and then do other classifications.
This is a very challenging area for data analysts. If you don’t know how to classify data reasonably, you won’t be able to draw conclusions when faced with a large amount of scattered data. When I tutor my classmates, I often find similar problems, so I simply made a guide on “How to Label” and put it in the Knowledge Planet for reference.
In-depth application of the people-cargo-field model
If we discuss the people, goods and places in depth, we will find more complex situations, such as:
Case 1: Excellent benchmarks cannot be copied. For example: It is found that Xiao Zhang in the sales team is very good, but Xiao Zhang has his own customer resources, and others do not have this condition. At this time, you can only find other ways.
Case 2: Short-term strategies are unsustainable. For example, in the short term, sales are driven by promotions (goods), but promotions cannot be done all the time. You can only analyze and see what other methods are available without promotions.
Case 3: Two dimensions influence each other. For example, a certain product is only popular in a certain region, which means we need to observe the combination of product X in region to judge sales.