The three elements of the big model are outdated, and electricity, servers, and steel are the key to success
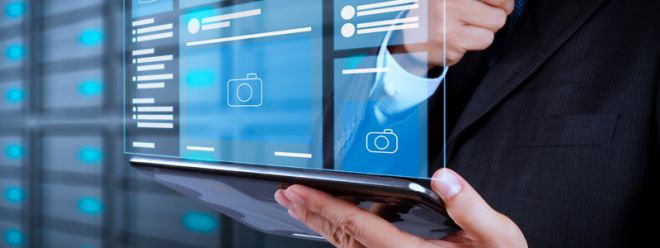
David Cahn, partner of Sequoia Capital, a well-known venture capital firm, participated in an interview with 20VC on the 5th. During the one-hour interview, this outstanding investor explained in detail the future macro trends of AI development.
Prior to becoming a partner at Sequoia Capital, David graduated from the University of Pennsylvania and served as General Partner and COO of Coatue, leading Coatue's investments in Runway, HuggingFace, Notion, and Supabase.
Next, let’s follow the footsteps of venture capital giants to see how Wall Street prophets grasp the general direction of AI, how they view the AI input-output ratio, and what the unicorns will roll in the second half?
AI Input-Output Game Theory
Recently, technology giants have begun to release their second-quarter earnings reports. During the technology earnings season, a question has emerged in the minds of Wall Street people: With so much investment, when will AI start to make money?
Since ChatGPT kicked off the AI arms race, tech giants have promised that the technology will revolutionize every industry, spending tens of billions of dollars on the data centers and semiconductors needed to run large AI models.
Compared to their grand vision, the products they’ve launched so far feel a little puny — there’s no clear path to monetization for the chatbot, the cost-saving measures are modest, and AI search seems to have an uncertain future.
But big tech companies have seen relatively little in the way of profitable new products powered by artificial intelligence despite spending billions, and investors are starting to get antsy.
For example, after investing heavily to adapt to the wave of artificial intelligence, Intel is now trying to control the situation by cutting $10 billion in costs and laying off tens of thousands of employees. The company's stock price plummeted 25% on Friday.
David wrote an article in September last year, "AI's $200B Question", the $200 billion problem of artificial intelligence.
Article address: https://www.sequoiacap.com/article/follow-the-gpusperspective/
Last month, David wrote another article titled "AI's $600B Question", the $600 billion question of artificial intelligence.
Article URL: https://www.sequoiacap.com/article/ais-600b-question/
From 200 billion to 600 billion, David quantified a very important indicator in these two articles, that is, there is a very large gap between the revenue expectations implied by AI infrastructure construction and the actual revenue growth in the AI ecosystem.
This gap is the $600 billion mentioned in the title of the article.
In the program, David believes that "AI changes the world" and "AI capital expenditure levels are too high" currently coexist.
In the past year, artificial intelligence has been like a dollar incinerator, although many supporters say, "No matter how high the number is, it doesn't matter. AI will change the world, don't worry."
However, David believes that we should not be blindly optimistic and should specifically quantify the input-output ratio of AI, which is why he wrote about the $600 billion problem of artificial intelligence.
On the one hand, we believe that artificial intelligence has a bright future; on the other hand, we must also clearly understand that capital expenditures on artificial intelligence will also be unlimited in the next two years.
You can believe in artificial intelligence, but you can also believe that the capital expenditure on artificial intelligence may be difficult to repay, at least in the next year.
During the show, David mentioned that he had just discussed this topic with Zuckerberg two days ago. Zuckerberg also recognized this problem. When he first entered the field of artificial intelligence, he tried to say that capital expenditures and budgets were not important.
But as the AI arms race enters its next phase, they all understand there are risks, but it’s a risk worth taking.
The capital market will cause a lot of "capital loss" when chasing technology hot spots. Speculators will accelerate the pace of technological progress, but a large number of speculators will fail in the market.
Therein lies the game theory behind AI investing. If AGI does arrive, it will be a great investment. But what if it doesn’t arrive? That’s the risk that investors and tech giants must take.
David believes that the current AI bubble is mainly concentrated in GPU, which is AI infrastructure, so Nvidia has emerged as the biggest winner.
But as technology develops, the price of computing power will surely drop, and the ultimate beneficiaries will shift from infrastructure creators to users.
Core: Data Center Infrastructure
David mentioned an interesting point in the program. He believes that the current development of AI is conducive to the development of start-ups.
Big tech giants are producers of computing, startups are consumers of computing.
So if there is a perceived overproduction of computing and the price of computing falls, then startups will profit from it.
Lower computing costs will translate into higher gross margins for startups, which directly means higher value.
In other words, if the giants succeed in investing in computing power, we will have amazing products and consumers will get huge value from them. If they fail, it’s not a loss, as we are not the ones who pay for it.
The host continued to ask questions about computing power. One is that computing is overproduced, which means that the cost of startups will decrease. And Altman once said very optimistically that computing power is the currency of the future. Are these two views contradictory?
David explained that although computing power sounds abstract, it is actually a bunch of physical things located in a data center somewhere, with a bunch of GPUs, a bunch of liquid cooling systems, and so on.
So David believes that the reason why the discussion around AI computing power has become somewhat contradictory is that the terms "computing" and "cloud" do not really capture the physical essence behind it.
The reality is that we don’t know how to build a GPU data center yet, so the big tech companies are left to build it in the best way possible.
Two years later, Nvidia's B100 chip becomes the dominant chip, so the issue of replacing the H100 chip arises, and the liquid cooling system will also replace the air cooling system on a large scale.
Therefore, David believes that in the next stage, construction efficiency may be more important than research breakthroughs. Technological breakthroughs allow models to become larger and larger, but there must also be data centers that are large enough to accommodate the models.
However, computing is generated from physical assets and faces huge construction costs and obsolescence costs.
David also mentioned a great quote he heard someone say recently, “No one will train a cutting-edge model twice in the same data center, because by the time you train it, the GPUs will be outdated and the data center will be too small.”
Assuming the model changes, assuming the Scaling Law continues to hold true, and AI continues to thrive in the future, that means the architecture of the data center needs to be changed and new chips are needed.
The bigger these models got, the more Scaling Law became dominant, all the researchers jumped from one lab to another, the data center became the most important asset, and every giant had to learn how to build these real data centers.
Today, seven top AI companies are at the starting line of this race to expand data center capacity: Microsoft/OpenAI, Amazon/Anthropic, Google, Meta, and xAI.
David analyzes how each player will take a unique approach (derived from their own business fundamentals) to win:
Meta and xAI are both consumer companies that will be vertically integrated, and both companies will seek to launch consumer applications supported by smarter models, simplifying model building and integrating it closely with data center design and construction.
Microsoft and Amazon have deep data center teams and deep pockets, and they’ve used those assets to form partnerships with top research labs.
They hope to monetize by selling training computing power to other companies on the one hand, and model inference on the other.
The two companies need to properly allocate resources between managing their cutting-edge models (GPT 5 and Claude 4) and building data centers.
Google has both consumer and cloud businesses, as well as its own internal research team. Google is also vertically integrated into the chip layer with TPU, and these factors should provide long-term structural advantages.
Three elements: steel, servers and electricity
Alexander Wang said on the show not long ago that he disagrees with many people. He believes that in addition to computing and computing power, data is the core bottleneck in the development of today's models and artificial intelligence.
David believes that computing models and data have been integrated, and it is difficult to say today that any big model company has a data advantage. Computing is just a commodity that needs to be paid for.
With capital expenditure plans in place and the competitive landscape defined, the second half of the AI race has begun. In this new phase of AI, steel, servers, and electricity will replace models, computing, and data as the “must-win” for anyone who wants to get ahead.
David said he was more interested in the industrial nature of AI, just like the industrial revolution that is taking place. Server-related chip innovations come from Nvidia, AMD and Broadcom. Nvidia has an amazing gross profit margin, and the competition will be very fierce. The chip war has just begun.
Secondly, regarding steel, there will be a large number of construction projects in the future, among which the biggest beneficiaries are basically construction companies and real estate companies.
Steel is a general term for all industrial goods, including generators and batteries. When David talked to the big cloud companies, they said they were calling manufacturers and saying they had a huge order.
They asked manufacturers to increase production capacity, but manufacturers hesitated because they would have to build new factories, which requires a lot of capital.
Finally, there is electricity. David thinks the electricity element will be a very interesting part. We have been waiting for the energy revolution for several years, and perhaps it will finally happen because of artificial intelligence.