How to integrate GPU cloud servers into AI infrastructure?
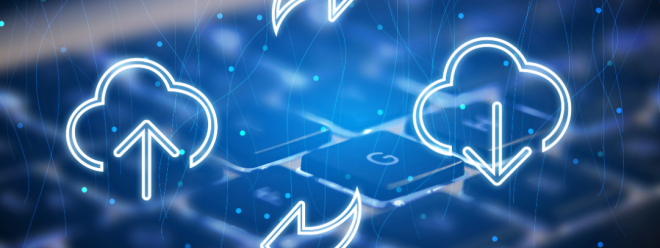
GPU cloud servers are cloud-based computing resources that utilize graphics processing units to handle high-performance tasks. Unlike traditional servers that rely solely on CPUs, GPU cloud servers are designed for parallel processing, making them ideal for compute-intensive applications such as machine learning and artificial intelligence.
In the B2B field, integrating GPU cloud servers into AI infrastructure has become a strategic move to improve performance and scalability. Machine learning models often require intense computing power, and GPU cloud servers provide a scalable solution that enables enterprises to process large data sets and run complex algorithms more efficiently. This capability is critical for businesses looking to maintain a competitive advantage in a rapidly evolving technology environment, as AI is driving innovation across industries. By integrating GPU cloud servers into their AI infrastructure, B2B enterprises can ensure they have the resources they need to effectively support their machine learning projects.
Benefits of GPU cloud servers for AI integration
Integrating GPU cloud servers into AI infrastructure brings many benefits to B2B enterprises. The main advantage is increased processing power. Graphics processing units are designed for parallel computing and can handle multiple tasks simultaneously. This capability is critical for machine learning applications, where large data sets and complex calculations are the norm.
Scalability is another important advantage. GPU cloud servers can easily scale to meet different workloads, providing the flexibility needed for AI projects with changing needs. This scalability is critical for businesses that need additional resources during peak times but don't want to use permanent infrastructure.
Deployment flexibility is also a key advantage. For example, with GPU cloud servers, enterprises can customize their cloud environment according to specific needs, whether it is deep learning, data analysis or AI model training. This adaptability helps enterprises optimize their AI infrastructure for maximum efficiency.
These advantages make GPU cloud servers ideal for B2B enterprises looking to enhance their AI infrastructure. By integrating these servers, enterprises can improve performance, increase scalability, and gain the flexibility they need to effectively support machine learning projects.
Assess AI infrastructure needs
When integrating GPU cloud servers into AI infrastructure, B2B enterprises must consider several key factors. Workload requirements are a major consideration—determine the amount of data and computational complexity your AI project requires. This will help evaluate the appropriate level of GPU cloud server resources required to maintain performance.
Scalability requirements are also critical. Consider whether the business will experience workload fluctuations and whether resources will need to be scaled quickly. GPU cloud servers provide flexibility, but you must ensure that the cloud provider can meet the scalability needs.
Cost constraints play an important role when evaluating AI infrastructure needs. Understand your budget and evaluate different pricing models to find cost-effective solutions. It's important to balance resource needs with financial considerations to avoid overcommitting cloud resources.
By considering these factors, B2B enterprises can make informed decisions about integrating GPU cloud servers into their AI infrastructure, ensuring they meet current and future needs without exceeding budget constraints.
Strategies for integrating GPU cloud servers into AI infrastructure
Integrating GPU cloud servers into AI infrastructure requires effective strategies to ensure seamless implementation. One approach is to adopt a hybrid cloud setup, where enterprises combine on-premises infrastructure with cloud-based resources. This strategy provides flexibility, allowing businesses to leverage existing hardware while benefiting from the scalability of the cloud.
Resource management is another key strategy. By carefully monitoring resource usage and employing technologies such as automatic scaling, enterprises can optimize cloud resource allocation. This helps maintain efficiency and reduces the risk of over-provisioning, resulting in cost savings.
Flexible deployment is also key to successful integration. GPU Cloud Server offers a variety of deployment options, allowing enterprises to tailor their infrastructure to meet specific AI project requirements. This flexibility extends to the choice of software frameworks and tools, allowing businesses to use the technology they prefer.
GPU cloud server scalability and flexibility
Scalability and flexibility are important components of AI infrastructure, especially for B2B enterprises with varying workload needs. GPU cloud servers provide scalable solutions, allowing enterprises to increase or decrease resources as needed. This flexibility is critical for businesses that require additional computing power during peak times without requiring permanent infrastructure investments.
The ability to dynamically scale resources means businesses can respond quickly to changes in demand. GPU cloud servers can automatically adjust to accommodate increased workloads, ensuring AI projects continue to run smoothly. This scalability helps companies maintain consistent performance during slower periods without overcommitting resources.
Flexibility isn't limited to scalability. GPU cloud servers offer a range of hardware and software configurations, enabling enterprises to customize their cloud environments. This adaptability enables enterprises to try different setups and find the configuration that works best for their AI projects.
By leveraging the scalability and flexibility of GPU cloud servers, B2B enterprises can create efficient and adaptable AI infrastructure that supports the changing needs of machine learning and AI projects.
Cost-effectiveness and pricing models
Cost-effectiveness is a key consideration when integrating GPU cloud servers into AI infrastructure. Different pricing models offer varying degrees of flexibility, allowing businesses to choose the most cost-effective option. Pay-as-you-go is a popular model that allows businesses to pay only for the resources they use. This approach is ideal for businesses with fluctuating workloads.
Subscription-based pricing offers a fixed rate for a specified period, providing stability and predictability for your budget. This model is beneficial for businesses with a stable workload because it allows them to plan their expenses more accurately. Reserved Instances are another cost-effective option that allow businesses to reserve computing resources at a discounted price.
Resource optimization technologies such as load balancing and autoscaling further improve cost efficiency. By evenly distributing workloads and scaling resources based on demand, enterprises can reduce unnecessary costs and maximize resource utilization.
Summarize
Integrating GPU cloud servers into AI infrastructure requires a strategic approach, including hybrid cloud setup, resource management, and flexible deployment. These strategies, combined with scalability and cost-effectiveness, enable B2B enterprises to build powerful AI environments. As AI and machine learning continue to evolve, GPU cloud servers will play a central role in driving innovation and shaping the future of the B2B industry.