OpenAI is revealed to be planning to build a “data market”
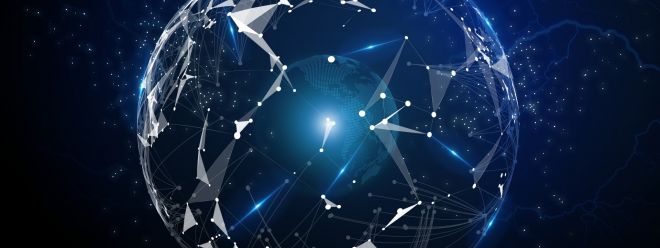
There is really no data available on the entire network!
Foreign media reported that companies such as OpenAl and Anthropic are working hard to find enough information to train the next generation of artificial intelligence models.
A few days ago, it was revealed that OpenAI and Microsoft are joining forces to build a supercomputing "Stargate" to solve computing power problems.
However, data is also the most important elixir for training the next generation of powerful models.
Faced with the data problem that exhausts the Internet, AI start-ups and major Internet companies really can’t sit still.
GPT-5 training, using YouTube videos
Whether it is the development of the next generation GPT-5 or powerful systems such as Gemini and Grok, it is necessary to learn from a large amount of ocean data.
Predictably, high-quality public data on the Internet has become very scarce.
At the same time, some data owners, such as institutions such as Reddit, have enacted policies to prevent AI companies from accessing the data.
Some executives and researchers say demand for high-quality text data could outstrip supply within two years, potentially slowing the development of artificial intelligence.
Including November 2022, researchers such as MIT warned that machine learning data sets may exhaust all "high-quality language data" before 2026.
Paper address: https://arxiv.org/pdf/2211.04325.pdf
WSJ reports that these artificial intelligence companies are looking for untapped sources of information and rethinking how to train advanced AI systems.
According to people familiar with the matter, OpenAI is already discussing how to train its next model GPT-5 by transcribing public YouTube videos.
In order to obtain more real data, OpenAI has also signed agreements with different institutions so that both parties can share some content and technology.
There are also companies that use synthetic data generated by AI as training materials.
However, this approach can actually cause serious malfunctions.
Previous research by Rice University and Stanford teams found that feeding AI-generated content to the model, especially after 5 iterations, will only lead to performance degradation.
Researchers gave an explanation for this, called "model autophagy disorder" (MAD).
Paper address: https://arxiv.org/abs/2307.01850
The use of AI-synthesized data is done secretly in these companies. This solution is already seen as a new competitive advantage.
AI researcher Ari Morcos said that "data shortage" is a cutting-edge research issue. He founded DatologyAI last year. Worked at Meta Platforms and Google’s DeepMind division.
His company builds tools that improve data selection and can help companies train AI models more cheaply.
"But there is currently no mature method to do this."
Data is scarce and becomes eternal
Data, computing power, and algorithms are all important resources for training powerful artificial intelligence.
For training large models such as ChatGPT and Gemini, they are completely based on text data obtained from the Internet, including scientific research, news reports and Wikipedia entries.
The material is divided into "chunks" - words and parts of words - and the model uses these chunks to learn how to form human-like expressions.
Generally speaking, the more data an AI model receives for training, the stronger its capabilities will be.
It was OpenAI's heavy investment in this strategy that made ChatGPT famous.
However, OpenAI has never disclosed the training details of GPT-4.
But Pablo Villalobos, a researcher at the research organization Epoch, estimates that GPT-4 was trained on as many as 12 trillion tokens.
He went on to say that based on the principle of Chinchilla's scaling law, if it continues to follow this expansion trajectory, AI systems like GPT-5 will require 60 trillion to 100 trillion tokens of data.
Taking advantage of all available high-quality language and image data may still leave a gap of 10 trillion to 20 trillion, or even more tokens, and it is unclear how to bridge this gap.
Two years ago, Villalobos wrote in a paper that there was a 50% chance that high-quality data would be in short supply by mid-2024. By 2026, the probability of supply exceeding demand will reach 90%.
However, they are now becoming more optimistic and estimate that this will be postponed to 2028.
Most online data are useless for AI training because they contain a large number of sentence fragments, contaminated data, etc., or do not add knowledge to the model.
Villalobos estimates that only a small portion of the Internet will be useful for model training, perhaps only 1/10 of the information collected by CommonCrawl.
Meanwhile, social media platforms, news publishers and other companies have been restricting AI companies from using their own platform data for artificial intelligence training because of concerns about fair compensation and other issues.
And the public is unwilling to hand over private conversation data (such as chat history on iMessage) to help train models.
However, Zuckerberg recently touted Meta's ability to capture data on its platform as a major advantage of Al's research efforts.
He publicly stated that Meta can mine hundreds of billions of publicly shared images and videos on its network, including Facebook and Instagram, which total exceeds most commonly used data sets.
Data selection tool startup DatologyAI uses a strategy called “lesson learning.”
In this strategy, data is fed into a language model in a specific sequence, with the hope that the AI will form smarter connections between concepts.
In a 2022 paper, Datalogy AI researcher Morcos and co-authors estimated that if the data were correct, the model could achieve the same results in half the time.
This has the potential to reduce the huge costs of training and running large generative AI systems.
However, other research so far shows that the "curriculum learning" approach is not effective.
Morcos said the team is tweaking this approach, which is deep learning’s dirtiest secret.
OpenAI Google wants to build a "data market"?
Altman revealed last year that the company was researching new methods of training models.
"I think we're at the end of the era of these giant models. We're going to find other ways to make them better."
OpenAI has also discussed creating a "data marketplace," people familiar with the matter said.
In this market, OpenAI could build a way to determine the contribution of each data point to the final trained model and pay the provider of that content.
The same idea has been discussed within Google.
Currently, researchers have been working hard to create such a system, and it is unclear whether a breakthrough will be found.
Executives have discussed using its automatic speech recognition tool Whisper to transcribe high-quality video and audio samples on the Internet, according to people familiar with the matter.
Some of this will be done via public YouTube videos, and some of the data has already been used to train GPT-4.
Next step, synthesize the data
Some companies are also trying to produce their own data.
Feeding AI-generated text is considered “inbreeding” in the field of computer science.
Such models often output meaningless content, which some researchers refer to as "model collapse."
Researchers at OpenAI and Anthropic are trying to avoid these problems by creating so-called higher-quality synthetic data.
In a recent interview, Anthropic's chief scientist Jared Kaplan said some types of synthetic data could be helpful. At the same time, OpenAI is also exploring the possibility of synthetic data.
Many people who study data problems are optimistic that solutions to the "data shortage" will eventually emerge.