DifFlow3D: New SOTA for scene flow estimation, the diffusion model has another success!
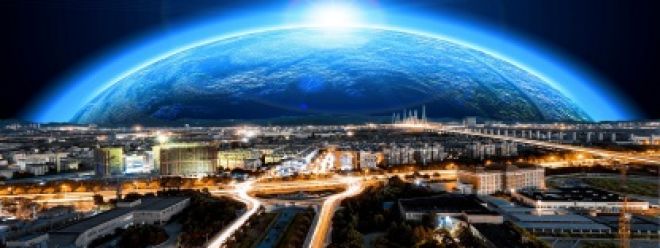
Scene flow estimation aims to predict the 3D displacement change of each point in a dynamic scene and is a basic task in the field of computer vision. However, previous works often suffer from unreliable correlations caused by locally constrained search ranges and accumulate inaccuracies in coarse-to-fine structures. To alleviate these problems, this paper proposes a novel uncertainty-aware scene flow estimation network (DifFlow3D), which adopts a diffusion probabilistic model. Iterative diffusion-based refinement is designed to enhance the robustness of correlation and have strong adaptability to difficult situations (such as dynamics, noisy input, repeated patterns, etc.). To limit the diversity of generation, three key flow-related features are exploited as conditions in our diffusion model. Furthermore, this paper develops an uncertainty estimation module in diffusion to evaluate the reliability of the estimated scene flow. DifFlow3D in this article achieves 6.7% and 19.1% reduction in three-dimensional endpoint errors (EPE3D) on the FlyingThings3D and KITTI 2015 data sets respectively, and achieves unprecedented millimeter-level accuracy on the KITTI data set (0.0089 meters for EPE3D). Additionally, our diffusion-based refinement paradigm can be easily integrated into existing scene flow networks as a plug-and-play module, significantly improving their estimation accuracy.
Main contributions:
To achieve robust scene flow estimation, this paper proposes a novel plug-and-play diffusion-based refinement process. To the best of our knowledge, this is the first time that a diffusion probabilistic model has been exploited in a scene flow task.
This paper designs a powerful conditional guidance to control the diversity of generation by combining coarse flow embeddings, geometry encoding, cross-frame cost volume.
In order to evaluate the reliability of our estimated flow and identify inaccurate point matches, we also introduce uncertainty estimates for each point in our diffusion model.
Our method outperforms all existing methods on both FlyingThings3D and KITTI datasets. In particular, DifFlow3D in this article achieves millimeter-level endpoint error (EPE3D) on the KITTI dataset for the first time. Compared with previous work, our method is more robust to challenging situations, such as noisy input, dynamic changes, etc.
Web design:
Scene flow, as a basic task in computer vision, refers to the estimation of a three-dimensional motion field from continuous images or point clouds. It provides information for low-level perception of dynamic scenes and has various downstream applications, such as autonomous driving [21], pose estimation [9] and motion segmentation [1]. Early work focused on using stereo [12] or RGB-D images [10] as input. With the increasing popularity of 3D sensors, such as lidar, recent work often takes point clouds directly as input.
As a pioneering work, FlowNet3D [16] uses PointNet++ [25] to extract hierarchical features and then iteratively regresses the scene flow. PointPWC [42] further improves it through pyramid, deformation and cost volume structures [31]. HALFlow [35] follows them and introduces an attention mechanism for better flow embedding. However, these regression-based works often suffer from unreliable correlation and local optima problems [17]. There are two main reasons: (1) In their network, K nearest neighbors (KNN) are used to search for point correspondences, which does not take into account correct but distant point pairs, and there is also matching noise [7] . (2) Another potential problem comes from the coarse-to-fine structure widely used in previous works [16, 35, 36, 42]. Basically, the initial flow is estimated at the coarsest layer and then refined iteratively in higher resolutions. However, the performance of flow refinement is highly dependent on the reliability of the initial coarse flow, since subsequent refinements are usually limited to a small spatial extent around the initialization.
In order to solve the problem of unreliability, 3DFlow [36] designed an all-to-all point collection module and added reverse verification. Similarly, Bi-PointFlowNet [4] and its extension MSBRN [5] propose a bidirectional network with forward-backward correlation. IHNet [38] utilizes a recurrent network with a high-resolution bootstrapping and resampling scheme. However, most of these networks suffer from computational cost due to their bidirectional correlations or loop iterations. This paper finds that diffusion models can also enhance the reliability of correlations and resilience to matching noise, thanks to their denoising nature (shown in Figure 1). Inspired by the discovery in [30] that injecting random noise helps jump out of the local optimum, this paper reconstructs the deterministic flow regression task using a probabilistic diffusion model, as shown in Figure 2. In addition, our method can be used as a plug-and-play module to serve the previous scene flow network, which is more general and adds almost no computational cost (Section 4.5).
However, leveraging generative models in our task is quite challenging due to the inherent generative diversity of diffusion models. Unlike the point cloud generation task that requires diverse output samples, scene flow prediction is a deterministic task that computes precise per-point motion vectors. To solve this problem, this paper utilizes strong conditional information to limit diversity and effectively control the generated flow. Specifically, a rough sparse scene flow is first initialized, and then flow residuals are generated iteratively through diffusion. In each diffusion-based refinement layer, we utilize coarse flow embedding, cost volume, and geometric encoding as conditions. In this case, diffusion is applied to actually learn a probabilistic mapping from conditional inputs to stream residuals.
Furthermore, little prior work has explored the confidence and reliability of scene flow estimation. However, as shown in Figure 1, dense flow matching is prone to errors in the presence of noise, dynamic changes, small objects, and repeated patterns. Therefore, it is very important to know whether each estimated point correspondence is reliable. Inspired by the recent success of uncertainty estimation in optical flow tasks [33], we propose point-wise uncertainty in the diffusion model to evaluate the reliability of our scene flow estimation.
image 3. The overall structure of DifFlow3D. This article first initializes a rough sparse scene flow in the bottom layer. Iterative diffusion refinement layers are then used in conjunction with flow-related conditional signals to recover denser flow residuals. To evaluate the reliability of the flows estimated in this paper, the uncertainty at each point will also be predicted jointly with the scene flow.
figure 2. Schematic diagram of the diffusion process used in this article for scene flow estimation.
Figure 4. Visualizing uncertainty. During the training process, the uncertainty interval designed in this article gradually shrinks, which promotes the predicted flow to move closer to the true value.
Experimental results:
figure 1. Comparison in challenging situations. DifFlow3D predicts uncertainty-aware scene flow using a diffusion model that is more robust to: (a) dynamic changes, (b) noisy inputs, (c) small objects, and (d) ) repeating pattern.
Figure 5. Visualization results without and with diffusion-based scene flow refinement (DSFR).
Figure 6. Add random Gaussian noise to the input points.
Figure 7. The role of uncertainty in the training process. This paper visualizes the uncertainty intervals at different training stages (10th round and 100th round).
Summarize:
This paper innovatively proposes a diffusion-based scene flow refinement network that is aware of estimation uncertainty. This paper adopts multi-scale diffusion refinement to generate fine-grained dense flow residuals. To improve the robustness of the estimation, this paper also introduces point-wise uncertainty jointly generated with the scene flow. Extensive experiments demonstrate the superiority and generalization ability of our DifFlow3D. It is worth noting that the diffusion-based refinement of this paper can be applied to previous works as a plug-and-play module and provide new implications for future research.