The future of deep learning: Trends and emerging technologies
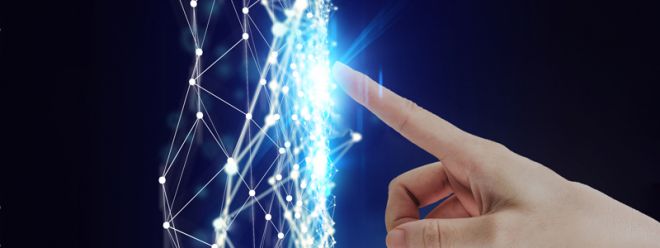
Deep learning, a subset of artificial intelligence (AI), continues to drive technological advancements and shape the way machines perceive, analyze and respond to data. This article will explore the latest trends and emerging technologies that will redefine the AI landscape in the coming years.
Exponential growth in model size
Taking models such as GPT-3 as an example, the trend of larger and larger neural network models demonstrates the drive for more complex and powerful artificial intelligence. The explosion in model size makes it possible to handle complex tasks, but also brings challenges in terms of computing resources and energy consumption.
Transfer learning and pre-trained models
Transfer learning, leveraging pre-trained models on massive data sets, is becoming a cornerstone of deep learning. This approach improves the efficiency of model training and promotes the application of deep learning in different fields, from healthcare to natural language processing.
Explainable Artificial Intelligence (XAI)
As AI systems become more complex, there is an increasing emphasis on making them interpretable and interpretable. Explainable Artificial Intelligence (XAI) aims to provide insights into the decision-making process of deep learning models, promoting trust and transparency in their applications, especially in critical areas such as healthcare and finance.
federated learning
As privacy issues become increasingly prominent, federated learning is emerging as a solution. This decentralized training approach allows models to be trained on multiple devices without exchanging raw data, solving privacy concerns while still benefiting from the collective intelligence of disparate data sets.
neuromorphic computing
Inspired by the structure of the human brain, neuromorphic computing is gaining more and more attention. This approach aims to build hardware that mimics the neural structure of the brain, thereby enabling more energy-efficient and brain-like processing, with potential applications in edge computing and sensory processing.
The evolution of generative adversarial networks (GAN)
GANs are known for generating real-world data and are currently being developed to new heights. Applications range from deepfake detection to content creation. The continued development of GANs is expected to make progress in generating high-quality synthetic data for training purposes.
Edge AI and on-device learning
The shift to edge AI involves processing data directly on the device, rather than relying solely on centralized servers. On-device learning reduces reliance on cloud services, providing the benefits of real-time processing, lower latency, and improved privacy.
Artificial Intelligence for Drug Discovery and Healthcare
Deep learning has made significant progress in drug discovery, genomics, and personalized medicine. The application of artificial intelligence in healthcare extends beyond diagnostics and has the potential to revolutionize the drug development process and enhance patient care through personalized treatment plans.
The impact of quantum computing
As quantum computing advances, it has the potential to revolutionize deep learning. Quantum algorithms can significantly speed up certain calculations, unlocking new possibilities for complex artificial intelligence tasks, including optimization problems and large-scale simulations.
Ethical AI and bias reduction
Addressing ethical issues and reducing bias in AI algorithms are key considerations going forward. Efforts to develop ethical AI frameworks and implement fairness in models will play a key role in shaping responsible AI practices.
Summarize
The future of deep learning is an exciting frontier full of promises and challenges. As trends evolve and breakthrough technologies emerge, the integration of deep learning into every aspect of our lives has the potential to revolutionize industries, enhance human-machine collaboration, and contribute to a future where artificial intelligence is not only