Hong Kong’s largest AI fraud case! Deepfake changed the face of "British CFO" and directly defrauded the company of 200 million
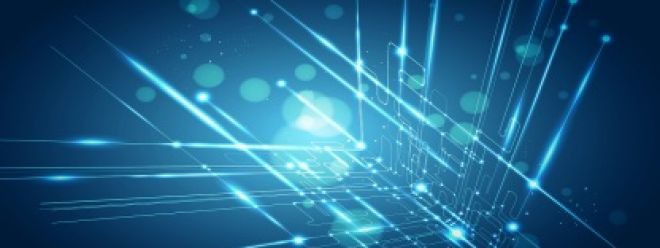
In the past few days, the ancient AI application - "AI face-changing" has broken out of the circle many times and has been frequently searched.
First, there were a large number of "AI nude photos" of Taylor Swift on the Internet, forcing X (formerly Twitter) to directly block all searches related to "Tylor Swift".
Today, the Hong Kong police announced an even more outrageous fraud case to the public:
The Hong Kong branch of a British multinational company was defrauded of HK$200 million by a scammer using fake "AI face-changing" and AI audio-synthesized video content to pretend to be the CFO of the head office!
The most advanced scams often only require the simplest AI technology
It is said that the employee of the Hong Kong branch received an email from the CFO of the British headquarters, saying that the headquarters was planning a "secret transaction" and needed to transfer company funds to several local accounts in Hong Kong for later use.
The employee did not believe the content of the email at first and thought it was a phishing scam email. But the scammer kept emailing him discussing the need for the secret deal, and eventually, made him a video call.
On the phone, the employee saw the company's CFO and "several other colleagues" he knew, all having a meeting together. The scammer also asked the employee to introduce himself during the video conference.
Then the "British leader" in the video conference asked him to transfer the money quickly, and then the video was suddenly interrupted. The employees who believed it was true remitted HK$200 million to five local accounts in Hong Kong in 15 times.
It was only after the whole incident lasted for five days that he came back to his senses and asked the British company for confirmation, and finally found out that he had been deceived.
The Hong Kong police stated in the report that past "AI face-changing" scams were usually one-on-one. This time, the scammer used a video conference call to create a fake "senior management team", which greatly increased the credibility.
Scammers used deepfake technology to successfully fake the image and voice of British company executives through the company's YouTube videos and other public media materials.
All participants in the video conference were real people, and then "AI" was used to change faces, replacing the face and voice of the scammer with the face and voice of the corresponding executive, and then issued instructions for transferring money.
In addition to the employee who was deceived, the scammer also contacted several other employees of the Hong Kong branch.
The police said that the entire case is still under further investigation and no suspects have been arrested, but they hope to release information to the public to prevent scammers from succeeding through similar methods again.
The police provided several ways to help identify whether it is an "AI face-changing" fraud.
First of all, if the other party mentions money in the video, you must be vigilant.
You can ask the other person to move their head and face quickly, and pay attention to see if there are any strange deformations in the picture.
Then ask some questions that only both parties know to verify the other party's true identity.
Banks are also deploying early warning systems for this type of fraud, and will issue reminders after discovering that users have made transactions with suspicious accounts.
After seeing this, foreign netizens lamented the power of Deepfake technology: Can I deepfake myself into a meeting so that I can sleep a little longer?
Some netizens also think that the whole story may have been made up by this "cheated employee", and he may have participated in the scam himself.
Origin of deepfake
Deepfake is essentially a special deep learning technology.
Originally originated from a Reddit user named "deepfakes". In December 2017, this user posted a face-swapping video of actresses such as Scarlett Johansson on the Reddit social networking site, making "Deepfake" synonymous with AI "AI face-swapping."
There is currently no generally accepted unified definition of Deepfake. The United States defines a "deep fake" in its "Malicious Falsification Prohibition Act of 2018" as "created or altered in such a way that a reasonable observer would mistakenly regard it as a true record of an individual's true words or actions." "audiovisual records", where "audiovisual records" refers to digital content such as images, videos and voices.
The core principle is to use algorithms such as generative adversarial networks or convolutional neural networks to "graft" the face of the target object onto the object being imitated.
Because the video is composed of consecutive pictures, you only need to replace the face in each picture to get a new video with changed faces.
First, the video of the imitating object is converted into a large number of pictures frame by frame, and then the face of the target imitating object is replaced with the face of the target object.
Finally, the replaced pictures are resynthesized into fake videos, and deep learning technology can automate this process.
With the development of deep learning technology, technologies such as autoencoders and generative adversarial networks have gradually been applied to deep forgery.
Deepfakes rely on a type of neural network called an autoencoder. They consist of an encoder that reduces the image to a lower-dimensional latent space, and a decoder that reconstructs the image from the latent representation.
Image source: Principles and practice of deep forgery
Deepfakes exploit this architecture by using a universal encoder to encode people into a latent space. The latent representation contains key features about their facial features and body posture.
It can then be decoded using a model trained specifically for the target. This means that the target's details will be superimposed on the underlying facial and body features of the original video and represented in the latent space.
Image source: Principles and practice of deep forgery
Another way to create deepfakes is to use so-called “generative adversarial networks” (Gan). Gan pits two artificial intelligence algorithms against each other.
The first algorithm, called the generator, takes in random noise and converts it into an image.
This synthetic image is then added to a stream of real images (such as celebrity images), which are fed into a second algorithm (called the discriminator).
At first, the composite image looked nothing like a human face. But by repeating this process countless times and providing performance feedback, both the discriminator and generator will improve.
Given enough cycles and feedback, the generator will start generating completely lifelike faces of completely non-existent celebrities.
Previously, Swift’s AI indecent photos were posted on the X platform
It went viral and even alarmed the White House spokesman.
At the White House press conference on January 26, spokesperson Karine Jean-Pierre was asked about the incident and said that the incident was alarming.
"While social media companies have their own independent decisions on content moderation, we believe they have an important role in strictly enforcing their regulatory regimes to prevent the spread of misinformation and intimate images without a person's consent."
How to identify and detect fake videos
As for the proliferation of Deepfake content, more and more research is focusing on identification and detection technologies.
Paper address: https://openaccess.thecvf.com/content/WACV2022/papers/Mazaheri_Detection_and_Localization_of_Facial_Expression_Manipulations_WACV_2022_paper.pdf
A new method developed in 2022 by a team of computer scientists at the University of California, Riverside, to detect manipulated facial expressions in deepfake videos. The method can detect these fake videos with up to 99% accuracy.
This method divides the task of detecting fake videos into two parts in a deep neural network.
The first branch identifies facial expressions while providing information about the region containing that expression.
These areas can include the mouth, eyes, forehead, etc.
This information is then fed into the second branch, which is an encoder-decoder architecture responsible for operation detection and localization.
The research team named this framework "Expression Manipulation Detection" (EMD), which can detect and locate specific areas in an image that have been altered.
Author Ghazal Mazaheri: "Multi-task learning can exploit salient features learned by facial expression recognition systems to benefit the training of traditional manipulation detection systems. This approach achieves impressive performance in facial expression manipulation detection,"
The researchers conducted experiments on two challenging facial manipulation datasets, and they demonstrated that EMD performed better in facial expression manipulation and identity exchange, accurately detecting 99% of tampered videos.
In the future, only by making efforts at both the technical and policy levels will it be possible to control the negative impact of Deepfake technology within a reasonable range.