Ten "pits" on the road to GenAI's success
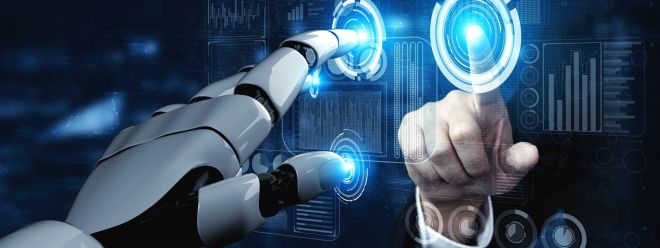
Want to implement generative artificial intelligence (GenAI)? Good news! Most IT decision-makers see the potential of this transformative technology, and you can consider yourself one of them. While GenAI has the potential to add significant efficiencies to the business, it also presents a set of challenges that must be overcome.
Here are the top ten challenges in implementing GenAI, ranked in descending order of importance.
1. Wrong data
The biggest challenge in implementing GenAI is bad data. If you can't confirm that the data is correct, the iterations from which it comes are carefully planned, and guaranteed to be safe and reliable, you'll be behind before you even begin.
Although we seem to be living in a brand new era, the age of artificial intelligence will make your wildest dreams come true! The old adage “garbage in, garbage out” still rings true.
Data is the core of GenAI, and data management may always be a challenge. But there are positive developments. Since the early days of the big data boom 15 years ago, companies have been trying to rationalize their data foundation so that they can build bigger and better things on top of it.
Investments in data management are now paying off for these companies, as they are well-positioned to take advantage of GenAI immediately due to its better-than-average data quality.
2. Legal and compliance matters
What you can and cannot legally use artificial intelligence to do is currently a matter of debate. New laws and regulations are being developed to limit the extent to which organizations can use AI, so we are in something of a gray area when it comes to commercial adoption of AI.
The EU is moving firmly towards a rather strict law. The new law, known as the AI Act, could ban the most dangerous forms of artificial intelligence, such as public facial recognition, and require companies to use it for other less intrusive but still potentially harmful uses. Get approval for things like using AI for recruiting or college admissions.
The United States is catching up with its European counterparts in regulating artificial intelligence, with President Joe Biden signing an executive order in October directing federal agencies to begin drafting regulations, which do not have the force of law.
This legal ambiguity has raised concerns among large companies, which are reluctant to invest large sums of money to implement an artificial intelligence technology for a wide range of external fields because the technology may be banned or strictly regulated soon after launch. . For this reason, many AI applications are targeted at internal users.
3. Insufficient computing power
Users not only need powerful GPUs to train GenAI models, but also need them for inference. Huge demand for high-end Nvidia GPUs far outstrips supply. This is great for large companies and Nvidia shareholders who have enough money to buy or rent GPUs in the cloud, but not so great for small and medium-sized companies and startups that need GPU time to implement GenAI.
The “big queue” for GPUs won’t ease anytime soon—certainly not until the first half of 2024. While Nvidia and its rivals are working hard to develop new chip designs to train and run LLMs (large language models) more efficiently, it will take time to design and put them into production.
Instead of running LLM, many companies are moving to running smaller language models that don't require the same huge resources as larger models. There are also efforts to reduce the size of LLM through compression and quantization.
4. Transparency and explainability
Transparency and explainability have been issues even before GenAI became a topic of discussion in corporate boardrooms. Just five years ago, companies were grappling with what to do with deep learning (DL), a subset of machine learning (ML) that uses neural network technology to find patterns in large amounts of data.
In many cases, companies choose to put into production systems based on simpler machine learning algorithms, even though deep learning yields greater accuracy, because they cannot explain how the deep learning system arrived at its answer.
The large language models (LLMs) that underpin GenAI are a form of neural networks that are, of course, trained on huge corpora of data — in the case of GPT-4, basically the entire public internet.
This poses a big problem when explaining how LLM gets its answer. There is no direct way to meet this challenge. Some methods have emerged, but they are somewhat complex. This remains an area of active research and exploration in academia, industry, and government R&D departments.
5. Accuracy and Illusion
No matter how good your GenAI application is, it has the potential to make something up, or, in the parlance of the field, "hallucinate." Some experts say it's normal for any artificial intelligence to hallucinate when asked to generate or create something that didn't exist before, such as a sentence or a painting.
While experts say hallucinations may never be completely eradicated, the good news is that rates have been declining. The GPT error rate of early versions of OpenAI was around 20%. This number is now estimated to be below 10%.
There are techniques to mitigate the tendency of AI models to hallucinate, such as by cross-checking the results of one AI model against another, which can reduce the hallucination rate to less than 1%. Mitigating hallucinations depends heavily on the actual use case, but it's something AI developers must keep in mind.
6. Lack of AI skills
As with any new technology, developers need a new set of skills to build GenAI, and it introduces many new technologies that developers must become familiar with.
For example, how to connect existing data sets to LLM and obtain relevant answers from them while meeting regulatory, ethical, security and privacy requirements requires some skills. A salary survey shows that IT engineers are emerging so quickly that they have become the highest-paid profession in the IT industry, with an average salary of more than $300,000.
In some ways, however, GenAI requires less high-end data science skills than previously required to build and implement AI applications, especially when using pre-built LLMs such as GPT-4. In these cases, some knowledge of Python is enough.
7. Security and privacy
GenAI applications work according to prompts. Without some type of input, you won't get any generated output. Without any controls, there's nothing to stop employees from prompting GenAI applications with sensitive data.
For example, a report released last June found that 15% of employees regularly pasted confidential data into ChatGPT. Many major companies, including Samsung, Apple, Accenture, Bank of America, JPMorgan Chase, Citigroup, Northrup Grumman, Verizon, Goldman Sachs, and Wells Fargo, have banned the use of ChatGPT within their companies.
Once data enters LLM, users have no guarantee where the data will come out. For example, OpenAI tells users that it uses their conversations to train its models. If you don't want your data to end up in your model, you'll need to purchase an enterprise license. Cybercriminals are becoming increasingly adept at stealing sensitive data from models. That's one of the reasons why data breaches feature on the Open Web Application Security Project's (OWASP) top 10 security risks.
Even if the data in the model itself is locked, other vulnerabilities exist. Through your IP address, browser settings and browsing history, GenAI apps may collect other information about you without your consent, including political beliefs, according to a VPN company called Private Internet Access or sexual orientation.
8. Ethical issues
Even before GenAI breaks out in late 2022, the field of artificial intelligence ethics is already developing rapidly. Now that GenAI has become the centerpiece of every businessman’s 2024 playbook, the importance of AI ethics has grown significantly.
Many companies are grappling with some of the larger issues related to implementing artificial intelligence, including how to deal with biased machine learning models, how to obtain consent, and how to ensure that models are transparent and fair. These are not trivial issues, which is why ethics remains a huge challenge.
Deloitte has been one of the industry leaders in thinking about the ethics of AI, creating the Trustworthy AI Framework back in 2020 to help guide ethical decision-making in AI. The guidance, led by Beena Ammanath, executive director of the Deloitte AI Institute, still applies to GenAI.
9. High cost
Executives must watch every penny spent on GenAI.
Depending on how your GenAI application is developed, cost may be a significant part of the equation. McKinsey divides GenAI costs into three types. Using a pre-built GenAI application will cost $500,000 to $2 million. The amount of user investment to fine-tune an existing LLM based on a specific use case will be between $2 million and $10 million. Manufacturers building a base model from scratch, such as OpenAI, will spend between $5 million and $200 million.
What is more important to note is that the cost of GPU is only the beginning of training LLM. In many cases, the hardware requirements for inference on the data on a trained LLM will exceed the hardware requirements for training it. There are also human factors to building GenAI applications, such as the time-consuming data labeling required, the time it takes to train people to master the skills, and so on.
10. Lack of performance commitment
When it comes to building and deploying artificial intelligence solutions, many executives are excited, but many are not so excited. A recent EY survey of technology leaders in financial services found that 36% of respondents cited a lack of clear support from leadership as the biggest barrier to AI adoption.
The potential returns on investment in GenAI are huge, but there are pitfalls to be aware of. A recent HFS Research survey found that for many, the ROI of GenAI remains uncertain, especially with rapidly changing pricing models.
GenAI adoption will surge in 2024 as businesses look to gain a competitive advantage. The companies that ultimately succeed will be those that overcome these obstacles moving forward and find ways to implement GenAI applications that are legal, safe, accurate, effective, and don't lead to bankruptcy.