What exactly is semantic communication?
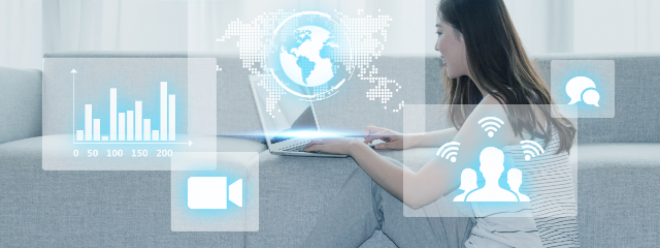
What exactly is semantic communication?
Everyone knows that since the outbreak of the information revolution, our information volume (data volume) has been expanding.
Text, pictures, audio, video... More and more data are being generated continuously, not only occupying our hard disk space, but also flooding the entire communication network.
These data make our work and life more convenient, and also promote the progress and development of society.
After entering the 21st century, under the joint stimulation of cloud computing, big data, Internet of Things and artificial intelligence, the growth trend of data has become more violent.
According to the IDC report, by 2025, the total amount of global data will reach 175ZB, which is approximately equal to 175 billion TB.
The ITU predicts that the annual growth rate of global mobile data traffic will reach 55% in 2030. The data traffic in 2030 will be 100 times that in 2020.
picture
Faced with such a huge increase in traffic, human beings' existing communication technology has been exhausted.
In 1948, the grandfather Claude Elwood Shannon published the classic paper "A Mathematics Theory of Communication", marking the birth of information theory.
Later, in 1949, he published "Communication in the Presence of Noise (Communication under Noise)", which clarified the basic problems of communication, gave the model of communication system, and the famous Shannon formula.
Since then, we have been conducting research on communication technology based on information theory and Shannon's formula.
After more than 70 years of accumulation, our communication technology has been infinitely close to the Shannon limit. The source coding technology represented by Huffman coding and algorithm coding compresses the source data to the extreme. The channel coding technology represented by LDPC code and polar code makes the best use of the channel.
Then, what should we do next? Facing the flood of data in the era of the Internet of Everything, our high-quality spectrum resources are getting less and less, and the cost of hardware and energy consumption is getting higher and higher. How should we deal with it?
Three Levels of Communication
Everyone may wish to calm down and think about it.
For a long time, our efforts in communication technology seem to be doing one thing - to send the symbols carrying information from the source to the destination completely, accurately and quickly.
It's like a hardworking courier whose sole mission is to deliver the goods entrusted to him by the sender to the recipient's hand intact and quickly.
picture
Then, when there are too many goods and the courier really can't carry them, will he have such a thought-is it really necessary to send all these goods?
picture
You may also have this experience:
You want to search for a good movie online. You picked one, spent a long time, and finally downloaded it, but when you opened it, you found that it was not your favorite at all. So, you can only delete it.
The hard work of data transmission done by the network has no value. Your time is also wasted.
Yes, here, we will think - what is the ultimate meaning of communication?
In fact, as early as the foundation of modern communication theory, the sages took this issue into consideration.
In 1938, the American philosopher Charles William Morris proposed the theory of symbols. He pointed out that symbols should include the triple concept of syntax-semantics-pragmatics.
After Claude Shannon proposed information theory, he and Warren Weaver (Warren Weaver) extended and perfected their own theories and models. They co-authored a book, still called "A Mathematical Theory of Communication".
Both of them realized then that the importance of semantics in communication. Therefore, they proposed three levels of communication, namely Level A/B/C.
picture
Level A: grammatical communication, solving technical problems, that is, how communication symbols ensure correct transmission;
Level B: Semantic communication, addressing semantic issues, i.e. how the sent symbols convey exact meaning;
Level C: Pragmatic communication, which addresses validity issues, i.e. how received meanings affect system behavior in desired ways.
For a long time, classical information theory has been limited to the level of grammatical information transmission, that is, Level A. In other words, we have been studying how to pass the data over.
Now, when traditional communication has entered a bottleneck, we can think about it—whether we can find a breakthrough in semantic communication.
Features of Semantic Communication
Semantic Communication (Semantic Communication) is a communication method that takes tasks as the main body and "understands first, then transmits".
It performs selective feature extraction, compression and transmission on the original signal, and then uses semantic level information for communication.
If we regard traditional communication as formal communication, then semantic communication is "expressive communication" and "content communication".
In other words: "Don't work stupidly, use your brain more."
What is the real purpose of communication is to let the other party understand what you mean. Speaking is just a way of expression. Words are meant to convey meaning. Then, there is no need to hold on to this sentence, but to see how to convey the meaning more efficiently.
From an academic point of view, reducing the receiver's uncertainty about the information, or in other words, reducing the received information entropy to 0, so that the receiver can correctly understand the sender's information content is "expressive" communication.
Everyone usually communicates and communicates, and you will also notice: For strangers, you need to give thousands of instructions to ensure that your meaning is understood. And for people you are close to, sometimes, a look is enough. isn't it?
"You know"
What is the difference between semantic communication and traditional source coding?
Traditional information source coding is the compression of information itself, which looks for the law of data and streamlines data through algorithms. Semantic communication, on the other hand, focuses on "understanding and digestion" and emphasizes "intelligence".
Architecture of Semantic Communication System
Semantic communication can significantly reduce data traffic and improve communication efficiency. So, how exactly does it work?
Semantic communication is still in the early research stage, and different research teams have different semantic communication architecture designs.
Moreover, there are different semantic communication models and architectures for different types of communication (text communication, image communication, audio and video communication, etc.) and communication for different purposes (whether there is a specific task or not).
An early model is to superimpose semantic communication on the traditional classical communication system.
picture
At the sending end, the information generated by the source is first sent to the semantic extraction module to generate a semantic representation sequence. Then, it is sent to the semantic information source encoder to compress and encode the semantic features. Then, it is sent to the channel encoder. Finally, enter the transmission channel.
At the receiving end, channel decoding is performed first, and then semantic decoding. The obtained semantic representation sequence is sent to the semantic recovery and reconstruction module, and finally the source data is obtained.
The channel part in the middle is realized by traditional classic communication.
Another model that is more representative now is source-channel joint coding. This approach is more holistic and holistic.
picture
You can see that, compared with traditional communication, semantic communication has an additional knowledge base. In fact, some models do not have a knowledge base, and are directly hardened on the semantic encoder.
More system models are based on knowledge bases. The performance and accuracy of the system model are highly dependent on the knowledge base.
A knowledge base is a bit like a codebook. Semantic communication cannot work properly if the knowledge bases at both ends are inconsistent.
The knowledge base is not like a codebook, which has fixed content and a single form. The knowledge base consists of many semantic knowledge graphs, which are divided into multiple levels, and can model entities, concepts, attributes and the relationship between them in the real world.
Based on the knowledge base, semantic understanding requires the "intelligence" we mentioned earlier.
Who is best suited for this job? AI, of course.
Simply put, it is to let AI complete the work of semantic understanding. The semantic encoding and decoding module is based on a knowledge base trained with massive data, and uses a deep learning network to fit semantic features, which can efficiently extract and reconstruct semantic information.
This is why, in the last ten years, semantic communication has begun to be mentioned again.
As early as 1956, French physicist L. Brillouin (L. Brillouin) pointed out that classical information theory ignores semantic communication because of engineering needs (the basic needs must be solved first), and it does not mean that people should always ignore semantic information .
Today, on the one hand, traditional information theory has encountered bottlenecks, and on the other hand, AI artificial intelligence technology is becoming more and more mature. Therefore, the time for us to revisit semantic communication is ripe.
It is particularly worth mentioning that AI artificial intelligence can help semantic communication, and in turn, semantic communication is also very suitable for the development of artificial intelligence.
Everyone should be able to understand: communication between subjects of the same type is often easy to simplify. Just like between people, communication is definitely simpler than between people and cattle.
In the future, we are developing in the direction of intelligence. After AI artificial intelligence is applied in batches, there will be many intelligent agents. There will be a lot of communication needs between these agents. Semantic communication itself is the "translation" of AI, and it will definitely have greater advantages for intelligent body communication.
Semantic Communication Challenges
The industry is unanimously optimistic about the development prospects of semantic communication. However, it is not easy to put this technology into practice and really play a role.
First of all, the basic theoretical system of semantic communication is not perfect.
Shannon's information theory laid the theoretical foundation for traditional grammatical information. He used a simple logarithmic formula to clearly define the information (entropy); and used the Shannon formula to delineate the channel capacity boundary of grammatical communication.
For semantic communication, no one has done these two important works. Compared with grammatical communication, semantic communication lacks rigorous mathematical representation and no solid theoretical basis.
The information measurement method of semantic communication is not particularly clear at present.
Traditional grammar communication has indicators such as bit error rate and packet loss rate, which are used to measure the quality of service. Semantic communication focuses on "expression" rather than "accurate transmission", so these indicators are useless.
In semantic communication, system performance evaluation can only be performed by macro task completion quality or semantic accuracy rate.
Speaking of accuracy, this is the second big problem with semantic communication.
With the current existing technology, even with the use of AI artificial intelligence, it is still impossible to achieve perfect accuracy. The recognition and restoration of semantics is much more difficult than imagined.
The third problem is the applicable scene problem.
Communication is a complex business. Data is diverse, including structured data and unstructured data. Text, pictures, audio and video, and some specific communication tasks are mixed together, and it is difficult to use a limited knowledge base for semantic extraction.
For example, if we use the knowledge base of industrial manufacturing scenarios to carry out semantic communication in agriculture, forestry, animal husbandry and fishery scenarios, it will definitely not work. However, how to accurately divide the boundaries of communication scenarios?
The knowledge base also touches on the fourth issue, which is security.
In reality, how to maintain two highly consistent knowledge bases? If the knowledge base is passed on, will it be leaked? How to ensure that the knowledge base is not invaded and disturbed?
All in all, there are still many challenges for semantic communication. These are all theoretical research problems, and there will be more problems if it is industrialized in the future.
Research Progress of Semantic Communication
As mentioned earlier, semantic communication is still in the early research stage. Since 2010, the research enthusiasm of this concept is getting higher and higher.
In China, many colleges and universities have built some semantic communication models and made initial progress.
The most representative one is Academician Zhang Ping of Beiyou and his team.
Around 2022, they proposed a new semantic information representation model - Semantic Base (Seb) in response to the evolution requirements of 6G.
Semantic base is the basic organizational unit of semantic information, similar to Shannon's bit in the traditional information theory system. It organizes information in a more structured, simplified, and flexible manner, and provides a new perspective for describing semantic information related to network intent.
They also proposed a 6G-oriented "one-side three-layer (semantic intelligence plane, semantic physical bearer layer, semantic network protocol layer, semantic application intent layer)" intelligent network protocol architecture, which provides an important reference for the study of semantic communication.
In addition to universities, some enterprises are also involved in the research and practice of semantic communication.
Taking China Mobile as an example, they have cooperated with Tsinghua University to develop a face-oriented scene-oriented conversational video semantic transmission solution, which is applied in China Mobile Ping An's rural network, and the effect is good.
Compared with traditional H.264 encoding, for face scenes, semantic communication reduces the bit rate to 10-20% under the same user experience, even if it is reduced to 3KB, a clear and smooth experience can be obtained.
epilogue
All in all, semantic communication technology has great research potential.
It is a major change in communication system design ideas and concepts, and it is likely to completely subvert our existing information and communication technology system.
Facing the future, whether semantic communication is a mule or a horse, let time tell us the answer.