Never pose like this in a room with WiFi because...
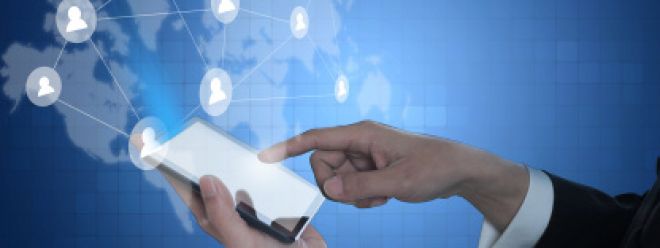
Never pose like this in a room with WiFi because...
This article is reprinted with the authorization of AI New Media Qubit (public account ID: QbitAI), please contact the source for reprinting.
Now, you can "see" what you're doing in your room using only WiFi ...
(What are you doing...ahhhh)
Multiplayer tracking is also so easy:
There is no need to take pictures or cameras during the process .
The input is only one-dimensional WiFi signal, and the output is three-dimensional human body posture.
Just two routers and you're done! The conversion cost is less than 500 yuan.
Moreover, it is not affected by ambient light and target occlusion, and the effect is close to the method of recognition based on 2D images.
Ah, does it mean that WiFi can "see" me? Taking it a step further... Can WiFi spy on me? ?
OMG, the Batman plot is going to be reflected in reality? ?
You must know that in "The Dark Knight", everyone's mobile phone in Gotham City has become a monitoring device, and every move of everyone in the same space can be recorded in real time.
Netizens have already conceived horror brain holes:
Imagine being able to see what our family is up to with just a TV connected to a WiFi receiver.
Some even say that in the future, you may have to wear protective coatings on your body to block WiFi signals.
Engage in full body tracking, don't use the camera
The method mentioned above is a new result of the Carnegie Mellon University (CMU) Robotics Institute.
The purpose of the research itself is to protect privacy. After all, monitoring is very necessary in many non-public places, such as nursing homes and homes for the elderly living alone, but it is difficult to guarantee privacy when using cameras.
The use of radar can solve the privacy problem, but the price and specific operability are very discouraging.
Therefore, the team thought of using the WiFi that is now necessary for almost every house to identify.
So on the device, only two ordinary home routers (each with at least 3 antennas) are needed.
The principle is also very simple, which is to use the channel state information (CSI) data in the WiFi signal.
The data is a bunch of complex decimal sequences that represent the ratio between transmitted and received signal waves.
As they travel between transmitter and receiver, they are modified once they come into contact with the human body.
Then, by interpreting these "changes", human posture can be detected.
To this end, the researchers developed a "region-based" convolutional neural network analysis pipeline that can localize various parts of the human body.
Then the phase and amplitude of the WiFi signal are mapped to the coordinates in the 24 human body regions to achieve the final whole body posture tracking.
Specifically, the model generates UV coordinates of the human body surface from WiFi signals through three components.
First, the original CSI signal is "cleaned" by the steps of amplitude and phase Sanitization.
Then, the processed CSI signal is converted into a 2D feature map through a two-branch encoder-decoder network.
Next, the 2D features are fed into an architecture called DensePose RCNN.
The architecture is inspired by DensePose, Facebook's open-source real-time human posture recognition system. DensePose was selected for the Oral session of CVPR in 2018, mainly to convert 2D images into 3D human body models.
So the purpose of this step is to calculate the 3D pose corresponding to the 2D feature map, that is, to estimate the UV coordinates.
Finally, before training the main network, the authors also minimized the difference between multi-level feature maps generated from images and those generated from WiFi signals, further refining the final results.
Although we can see from the naked eye that the final results of the two methods are similar, in terms of data, the image-based method works better.
For example, under the same environment layout, the accuracy of the WiFi-based method is lower than that of the image method.
△ Higher value means better
The same is true for different environment layouts.
At the same time, if an action not included in the data set is encountered, the method will not be able to recognize it successfully. If there are more than 3 people, there will also be a "shame" situation.
The two pictures on the left in the figure below are cases of rare action failures, and the two pictures on the right are cases of recognition failures involving more than 3 people.
However, the team believes that the above problems can be solved by further expanding the data set.
In addition, this method has high requirements on the placement of the router and will affect other WiFi networks.
From the CMU team, there are 2 Chinese authors
Paper one is Jiaqi Geng , who is from Carnegie Mellon University and received a master's degree in robotics in August last year.
△Jiaqi Geng
Another Chinese author is Dong Huang , who is now a senior project scientist at Carnegie Mellon University.
△Dong Huang
His research direction has been using deep learning for signal recognition. For example, real-time recognition of 2D human body poses using WiFi signals has been realized before.
The last author is Fernando De la Torre , who is now an associate professor at Carnegie Mellon University's Robotics Institute.
△Fernando De la Torre
His research direction is mainly computer vision, involving fields including human gesture recognition, AR/VR, etc.
In 2014, he founded FacioMetrics LLC, a company that developed face recognition technology, which was acquired by Facebook two years later.
The author team said that the current performance of the method is still limited by the small amount of data available for training. In the future, they plan to expand the data set.
Paper address: https://arxiv.org/abs/2301.00250