Get ready for Industry 4.0 with 5G and native AI
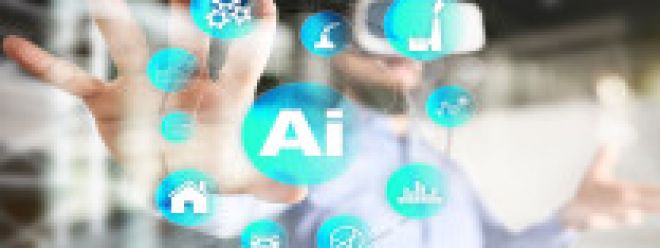
The Internet of Things (IoT) is a major business opportunity for mobile operators. That's why 5G was designed from the ground up to support demanding IoT use cases and requirements—including those that 4G and Wi-Fi struggled to handle.
For example, 5G has three feature sets that are well suited for Industry 4.0 applications. These include Ultra Reliable Low Latency Communication (URLLC), which reduces latency to as low as one millisecond, which is critical for mission-critical use cases such as Time Sensitive Networking (TSN) and Massive Machine Type Communication (mMTC), making 5G networks Capable of supporting up to 1 million IoT devices per square kilometer, such as autonomous material handlers, industrial robots and sensors. The final feature set is Enhanced Mobile Broadband ( eMBB), which enables bandwidth-intensive applications such as 4K cameras in and around factories to monitor production, employee safety and personal safety.
Even with these and other advanced capabilities, mobile operators will need additional tools to ensure their 5G networks can take full advantage of IoT opportunities. The same goes for operators of private 5G networks, with 76% planning to deploy these networks by 2024, according to a survey by Analysys Mason.
Beyond Baseline 5G
Artificial intelligence and machine learning (AI/ML) lay the foundation for Industry 4.0 and the benefits it brings. Mission-critical use cases in Industry 4.0 require hyper-real-time AI/ML performance. So 5G can't just enable artificial intelligence .It has to be AI native .
AI-native refers to building AI/ML capabilities into 5G network capabilities. An example of this is the integration of AI/ML to enhance the Radio Access Network (RAN) Media Access Control (MAC) scheduler. With this capability, the network can intelligently improve quality of experience (QoE) through hyper-real-time predictive analytics. QoE is critical for use cases such as smart manufacturing, where technologies such as digital twins can provide remote human assistance through immersive experiences to improve shop floor performance and enhance safety .
More importantly, this AI/ML enhancement also does not have to lock operators into proprietary ecosystems and suffer from vendor lock-in. Operators can benefit from Open RAN (O-RAN) guidelines, providing more choice and flexibility to maximize spectral efficiency. This flexibility allows them to overcome one of the main limitations of today's RAN architecture: radio resource management can be static and cannot adapt quickly to changing traffic conditions and user behavior.
This example also highlights how AI/ML will fundamentally change 5G communication systems and how they will be designed and deployed in the future. AI/ML can even control some physical (PHY) and media access control (MAC) layer functions. All of this directly benefits IoT, numerous Industry 4.0 use cases, and more - now and in the future.
Optimize efficiency and performance
Many manufacturing IoT applications are designed to maximize efficiency. Just as AI/ML is changing 5G networks, it can do the same for manufacturing IoT applications. Here, it can reduce the amount of radio resources, power and spectrum required. Of course, the electricity saved can help operators improve their green credentials and save on CAPEX and OPEX. For example, in the case of public 5G networks, the CAPEX and OPEX savings can help operators price their services competitively, yet profitably—a big part of the notoriously cost -sensitive IoT market Advantage.
These benefits are possible even in demanding use cases. For example, when IoT applications such as real-time 4K video require ultra-fast gigabit speeds, they do not require more radio resources, spectrum or power than low-bandwidth applications. How is this possible?
This is because RAN functions such as access control, radio resource scheduling, mobility management and radio resource management are currently rule-based. They cannot adapt to the dynamics of the network, or the services that every IoT device accesses. When ML-introduced intelligent automation powers RAN functions, especially in lower protocol layers, it can dynamically make decisions for efficient network resource allocation and improved user experience. As a result, it can dynamically boost use cases such as real-time 4K video.
Key Considerations When Infusing AI/ML
A key challenge when introducing ML into the lower RAN layers is the limited time window available for scheduling decisions, which takes about 1 millisecond. For IoT applications where every second counts, machine learning algorithms cannot introduce any latency. Even a few seconds of processing delay can severely impact overall QoE and destroy spectral efficiency.
It is also important to ensure that ML-based updates can run on existing 5G-gNodeB platforms without hardware accelerators or GPUs. This can be achieved by introducing intelligence into the most critical and time-sensitive Layer 2 MAC scheduler functions, which play a key role in radio resource allocation. In a lab setting, this approach improves spectral efficiency by 15% while increasing cell throughput by 11.76%. This is what enables operators (public and private) to dynamically increase the QoE of each IoT application. This is an amazing ability.
At the MAC layer, AI-driven predictive analytics can predict and then assign appropriate modulation and coding scheme (MCS) values to signal transmissions based on the signal quality and movement patterns of each IoT device. The RAN can then intelligently allocate MAC resources across the different network layers to meet each customized requirement. For example, it can provide more accurate MCS predictions, achieve better spectral efficiency, and intelligently handle different devices with different needs. This automation can effectively increase traffic per cell, enabling operators to manage more subscribers and successfully launch new IoT applications.
Robust, flexible, greener framework
The framework for native AI and 5G uses a fully containerized architecture. It is cloud-native and operates as a collection of independent and loosely coupled microservices. They are highly scalable and can run on top of public, private and hybrid clouds, giving operators more They use a service mesh architecture for managed, observable, and secure communications. By abstracting the complexities of a network of microservices using APIs and a service mesh, this powerful framework allows operators and enterprises to rapidly introduce and scale new services.
With both the hardware layer and the AI layer abstracted, manufacturers and other businesses have the agility, performance, and savings they need to stay connected, competitive, and profitable. Connectivity to native AI is critical to industry digitization and accelerating Industry 4.0 initiatives . The AI-native approach optimizes the radio resources allocated to users through intelligent link adaptation. This improves QoE and supports mission critical operations. Improved QoE is one of the key attributes of O-RAN. O-RAN and native AI are a powerful combination .
One of the key benefits of native AI and 5G O-RAN is particularly timely. Together, they can provide excellent QoE for Industry 4.0 through optimized radio resources and higher bandwidth. Importantly, it can do so without consuming more radio resources. That is, the power consumption for transmitting radio signals is lower. In short, this increases energy efficiency and enhances sustainability. Sustainability is seen at the top of the telco's agenda after research showed the industry's CO2 emissions were almost double that of aviation. Clearly, native AI on the mobile web isn't fast enough.